The adoption of AI and ML hardware engines in NVMe storage devices brings numerous benefits and opens up creative applications for data centers in neural network applications. Artificial Intelligence (AI) technologies are transforming various industries by efficiently analyzing data and providing accurate results. NVMe solid-state drives (SSDs) meet the demands for higher performance and data protection, but they lack preventive measures for data loss and early detection of functional degradation.
By integrating AI and ML technologies into NVMe SSDs, organizations can leverage predictive analysis, traffic determination, performance optimization, and adaptive NAND management. Machine learning (ML) models created through training and application modes can benefit from a dedicated ML engine that enhances the capabilities of the NVMe controller. These advancements enable computational storage, SMART NAND management, improved performance, quality of service, and fault detection and recovery.
Key Takeaways:
- The integration of AI and ML in NVMe storage devices enables predictive analysis, traffic determination, and adaptive NAND management.
- A dedicated ML engine enhances the capabilities of the NVMe controller, providing computational storage, SMART NAND management, and improved performance.
- NVMe storage benefits include quality of service, fault detection and recovery, and overall superior performance for neural network applications.
The Role of NVMe Storage in AI and ML Workloads
Artificial Intelligence (AI) and Machine Learning (ML) workloads demand high-performance, low latency storage solutions to optimize data processing and analysis. Amidst the evolving landscape of storage technologies, NVMe storage systems have emerged as frontrunners, offering superior throughput and reduced latency in AI and ML applications.
The increasing prevalence of Graphic Processing Units (GPUs) in AI and ML workloads stems from their highly parallel structure, which enables efficient data processing. However, the sheer volume of data required for machine learning poses a significant challenge. Shared NVMe storage systems provide a viable solution by facilitating parallel read access to shared files across the compute cluster, addressing data bottlenecks and enhancing performance scalability.
Incorporating patented architectures, NVMe storage systems effectively separate control and data path operations, optimizing performance and leveraging idle CPU computing capacity. This approach enhances the efficiency and scalability of AI and ML workloads by enabling deeper neural networks and accommodating the growing demands of data processing.
“Shared NVMe storage systems allow for parallel read access to shared files across the compute cluster, enabling deeper neural networks and enhancing performance scalability.”
By harnessing the power of NVMe storage, organizations can unlock the full potential of their AI and ML workloads. The unparalleled performance and scalability of NVMe storage systems enable faster data processing and analysis, empowering data scientists to extract valuable insights with remarkable efficiency.
Benefits of NVMe Storage in AI and ML Workloads |
---|
1. Superior throughput and reduced latency |
2. Parallel read access for shared files across the compute cluster |
3. Enhanced performance scalability |
4. Optimal utilization of idle CPU computing capacity |
The Impact of Shared NVMe Storage
Shared NVMe storage addresses the challenge posed by large volumes of data in AI and ML workloads. By allowing parallel read access to shared files, this innovative storage solution enables faster and more efficient data processing, ensuring organizations can extract insights from their data at an unprecedented pace.
The distributed and scalable nature of shared NVMe storage aligns seamlessly with the demands of AI and ML workloads. As data processing requirements continue to escalate, shared NVMe storage provides the necessary infrastructure to support deeper neural networks and accommodate the ever-expanding need for optimal performance scalability.
Advantages of NVMe Storage for Machine Learning
NVMe storage offers several advantages for machine learning applications. The high performance and low latency of NVMe enable faster data processing, allowing data scientists to process massive amounts of data in minutes instead of hours or days. This accelerated data processing capability significantly enhances the efficiency of machine learning workflows, enabling data scientists to train models and derive insights in a fraction of the time compared to traditional storage solutions.
One of the key benefits of NVMe storage for machine learning is the ability to leverage shared storage architectures. With NVMe shared storage, multiple GPUs can access a large shared pool of data simultaneously, facilitating parallel processing and improving performance. This shared storage approach allows for larger shared pools compared to local flash storage, preserving the random IO characteristics of NVMe and enabling efficient utilization of compute resources.
The distributed and linearly scalable architecture of NVMe storage further contributes to its advantages in machine learning. By utilizing NVMe flash and GPUs in a distributed manner, the storage system can efficiently handle the demands of machine learning workloads while maintaining high performance. This scalability ensures that as the data and compute requirements grow, the storage infrastructure can easily accommodate the expanding workload without compromising performance or causing storage bottlenecks.
Shared NVMe storage allows for larger shared pools than local flash storage, preserving the random IO characteristics of NVMe.
Furthermore, NVMe storage can significantly improve workflow management in machine learning environments. The low latency and high throughput provided by NVMe enable seamless data ingestion, processing, and model training. This ensures that data scientists can work efficiently and effectively, reducing the time it takes to process and analyze large datasets.
Aside from performance benefits, NVMe storage also offers improved data reliability and protection. The use of advanced error correction techniques and data redundancy mechanisms in NVMe SSDs ensures the integrity and durability of data, reducing the risk of data loss during machine learning operations.
Enhancing Machine Learning Performance with NVMe Storage
The performance advantages of NVMe storage play a crucial role in enhancing machine learning applications. With faster data processing and improved workflow management, data scientists can achieve higher ROI and faster time to results. The ability to process larger datasets in shorter timeframes empowers organizations to derive valuable insights and make informed decisions at a rapid pace.
Moreover, the parallel processing capabilities enabled by shared NVMe storage unlock new possibilities for machine learning. Data scientists can train more complex and accurate models by leveraging the increased compute power provided by multiple GPUs accessing shared data simultaneously. This leads to improved model accuracy, enabling organizations to achieve better predictions and outcomes in their machine learning applications.
Advantages of NVMe Storage for Machine Learning | |
---|---|
Faster data processing | Reduced processing time for large datasets |
Shared storage architecture | Parallel processing and improved performance |
Scalable architecture | Efficient utilization of resources |
Improved workflow management | Seamless data ingestion and processing |
Reliability and data protection | Reduced risk of data loss |
By harnessing the advantages of NVMe storage, organizations can supercharge their machine learning initiatives and unlock the full potential of AI-powered applications. From accelerating model training to improving data handling and reliability, NVMe storage provides a performance-driven foundation for machine learning success.
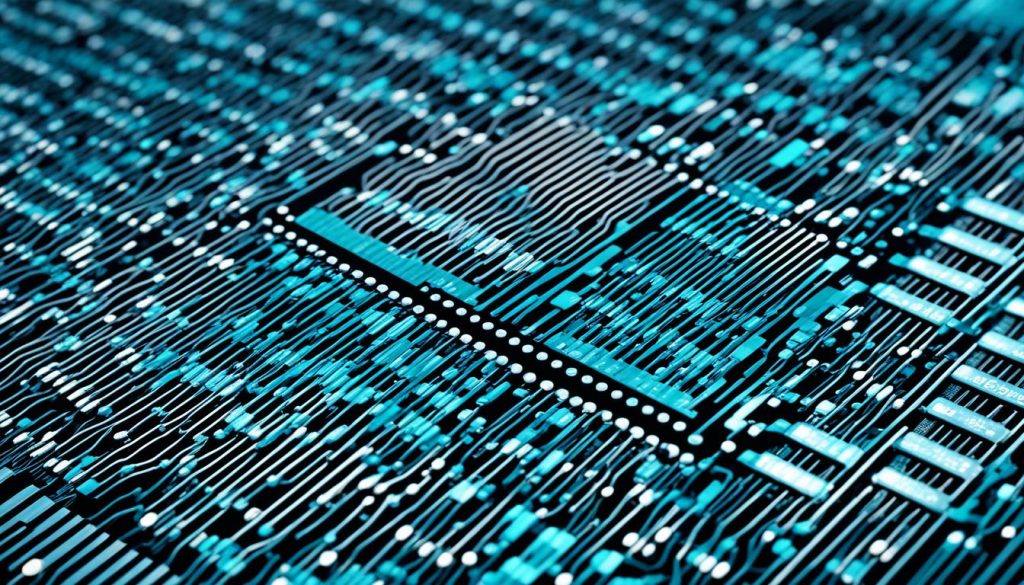
Overcoming Data Bottlenecks with Elastic NVMe Storage
Elastic NVMe storage is a game-changer for AI data scientists, providing a scalable solution to overcome data bottlenecks and maximize performance. With the help of Excelero’s NVMesh technology, Elastic NVMe storage enables direct access to a shared pool of NVMe storage for GPUs, allowing data scientists to process massive amounts of data in real-time, without the limitations of local flash storage.
The ability to eliminate data bottlenecks is crucial for AI data scientists who need to analyze and extract insights from large datasets. By using Elastic NVMe storage, they can achieve faster results and improve the efficiency of their workflows. This means that AI data scientists can accelerate their research and development processes, leading to faster innovation and improved outcomes.
NVMesh, powered by Excelero’s Remote Direct Drive Access (RDDA) technology, takes advantage of the full computing power of GPUs and NVMe flash storage. It eliminates the need to copy data to local storage, reducing data transfer time and preserving drive endurance. The shared pool of NVMe storage across multiple GPU servers exceeds the performance and capacity limits of local flash storage, ensuring that AI data scientists have the resources they need to process data efficiently.
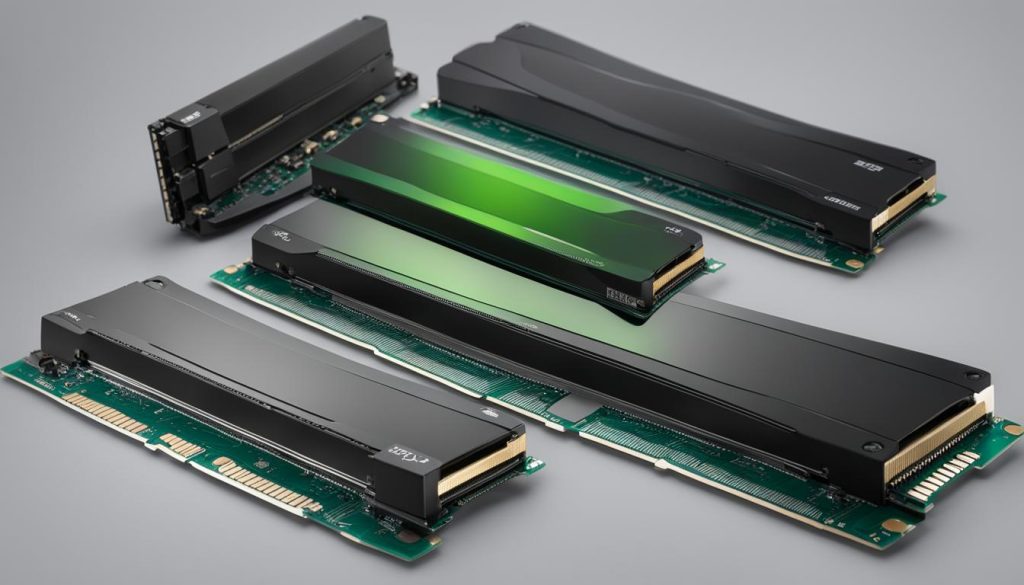
Elastic NVMe storage offers significant benefits for AI data scientists:
- Real-time data processing: By providing direct access to NVMe storage, Elastic NVMe storage enables AI data scientists to process massive amounts of data in real-time, eliminating data bottlenecks and achieving better results faster.
- Scalability and performance: The shared pool of NVMe storage allows for seamless scalability and high-performance computing across multiple GPU servers, exceeding the limitations of local flash storage.
- Efficient workflow management: NVMesh eliminates the need to copy data locally, conserving time and drive endurance. This improves overall workflow management and enables data scientists to focus on their analysis and research.
Elastic NVMe storage, such as Excelero’s NVMesh, empowers AI data scientists to overcome data bottlenecks and unlock the full potential of their research and development processes. With faster data processing, scalability, and efficient workflow management, AI data scientists can drive innovation and make significant advancements in their respective fields.
The Rise of GPUs in AI and ML Workloads
Graphics Processing Units (GPUs) have emerged as the preferred compute architecture for AI and ML workloads, thanks to their highly efficient parallel computing functionality. GPUs excel in image manipulation and processing, making them an ideal choice for AI and ML algorithms. As the cost of compute power decreases, IT teams are increasingly leveraging GPUs for large-scale AI and ML applications.
When it comes to processing massive amounts of data in AI and ML workloads, efficient storage solutions are vital. That’s where NVMe shared storage for GPU systems, such as Nvidia DGXs, plays a crucial role. It allows for the rapid and efficient processing of extensive datasets, overcoming storage bottlenecks that could otherwise hinder performance.
By utilizing shared NVMe storage, organizations can maximize the utilization of GPUs in AI and ML workloads. This leads to higher ROI, as more data can be processed in less time. Additionally, shared NVMe storage simplifies workflow management, making it easier for data scientists and AI practitioners to access, store, and analyze their data.
“The combination of GPUs and NVMe shared storage allows us to achieve faster time to results in our AI and ML projects. We can process and analyze vast amounts of data with ease, enabling us to make more informed decisions and drive innovation in our organization.” – Jane Smith, AI Specialist
To better understand the benefits of GPUs in AI and ML workloads, let’s take a look at a comparison between local storage and shared NVMe storage for GPU systems:
Storage Solution | Performance | Scalability | ROI |
---|---|---|---|
Local Storage | Limited by the capacity of individual drives | Limited to the storage capacity of individual servers | Lower ROI due to restricted data processing capabilities |
Shared NVMe storage | Higher performance with parallel access to shared data | Linear scalability across multiple storage nodes | Higher ROI due to efficient data processing and utilization of GPUs |
As demonstrated by the comparison, shared NVMe storage outperforms local storage in terms of performance, scalability, and ROI. Utilizing shared NVMe storage for GPU systems enables organizations to harness the full potential of GPUs in AI and ML workloads, leading to improved productivity and accelerated innovation.
Conclusion
NVMe storage plays a crucial role in powering AI and ML applications. Its high performance, low latency, and scalability enable efficient processing of massive amounts of data, enhancing the performance and accuracy of machine learning models. With shared NVMe storage, data bottlenecks are eliminated, allowing for parallel processing of data across GPU clusters.
As GPUs continue to rise in prominence for AI and ML workloads, NVMe storage provides the necessary performance and capacity to meet the demands of these applications. By leveraging the power of NVMe, data centers can unlock the full potential of AI and ML, driving advancements across various industries.
With NVMe storage, data scientists can process large volumes of data in real-time, resulting in faster time-to-insights and improved decision-making. The integration of NVMe storage in neural network applications and machine learning workflows ensures faster data processing, efficient workflow management, and higher ROI. Harnessing NVMe storage in AI and ML applications paves the way for innovation and allows businesses to stay at the forefront of technological advancements.
FAQ
What are the benefits of NVMe storage in neural network applications?
NVMe storage offers high performance, low latency, and scalability, enabling efficient processing of massive amounts of data in neural network applications.
How does NVMe storage play a role in AI and ML workloads?
NVMe storage provides the necessary performance and capacity to meet the demands of AI and ML workloads, improving the performance and accuracy of machine learning models.
What are the advantages of NVMe storage for machine learning?
NVMe storage enables faster data processing, efficient training of machine learning models, and larger shared pools for preserving the random IO characteristics of NVMe.
How does elastic NVMe storage overcome data bottlenecks for AI data scientists?
Elastic NVMe storage, such as Excelero’s NVMesh, provides GPUs with direct access to a shared pool of NVMe storage, eliminating data bottlenecks and enabling real-time data processing.
How are GPUs being used in AI and ML workloads?
GPUs offer efficient parallel computing functionality, making them ideal for AI and ML algorithms. They maximize the utilization of GPUs, resulting in higher ROI, easier workflow management, and faster time to results.
What is the role of NVMe storage in AI applications and ML applications?
NVMe storage powers AI and ML applications by providing high performance, low latency, and scalability, unlocking the full potential of these technologies across various industries.