Edge computing is revolutionizing the way businesses handle data processing and analysis. By bringing storage and compute resources closer to the data source, edge computing enables real-time insights and improved efficiency in decision-making. This is particularly significant in the age of artificial intelligence (AI) and the virtual flood of data generated by sensors and IoT devices.
In traditional centralized data centers, raw data is transmitted from client endpoints, across wide-area networks (WAN), and through corporate local-area networks (LAN) for processing and analysis. This process often leads to latency and bandwidth limitations, slowing down decision-making processes. However, with edge computing, data is processed at the edge, where it is generated. This eliminates the need to transmit large amounts of raw data, resulting in faster data processing and analysis.
Edge computing’s integration with AI takes data processing capabilities to a new level. AI algorithms and models can be deployed directly on local edge devices, such as sensors or IoT devices. This allows for faster processing speeds and real-time issue detection. The seamless integration of edge computing, AI, and 5G networks enables the coherent processing of massive amounts of data generated by IoT devices in various industries, including healthcare, manufacturing, autonomous vehicles, and smart cities.
With reduced latency and real-time decision-making, edge computing brings numerous benefits to industries. It enhances operational efficiency, minimizes downtime, and improves overall productivity. From industrial automation and predictive maintenance to route optimization in smart cities, edge computing opens doors to innovative use cases and transforms industries.
Edge computing, cloud computing, and fog computing are closely related concepts. While edge computing brings storage and compute resources closer to the data source, cloud computing relies on centralized data centers. Fog computing operates within a specific environment, utilizing fog node deployments for data processing and analysis. Each of these approaches has its own unique characteristics and applications in the distributed computing landscape.
Key Takeaways:
- Edge computing brings storage and compute resources closer to the data source, enabling real-time insights and improved efficiency in decision-making.
- AI integration with edge computing revolutionizes data processing by deploying AI algorithms directly on local edge devices.
- Edge computing benefits various industries, including healthcare, manufacturing, autonomous vehicles, and smart cities, by enhancing operational efficiency and enabling real-time optimization.
- Edge computing, cloud computing, and fog computing are related but have distinct characteristics and applications in the distributed computing landscape.
- The future of data centers and AI-driven innovations will be shaped by the advancements in edge computing technology.
What is Edge Computing?
Edge computing architecture is a decentralized approach that moves storage and compute resources out of the centralized data center and closer to the source of data. Unlike traditional data processing methods, edge computing eliminates the need to transmit raw data to a central data center for processing and analysis. Instead, data is processed at the edge, where it is generated, allowing for faster data processing and analysis.
By deploying edge computing architecture, businesses can harness real-time business insights and make informed decisions based on the data collected from multiple sources. This proximity to the data source enables organizations to obtain real-time insights and predictions, such as equipment maintenance predictions, that can drive operational efficiency and provide a competitive edge.
“Edge computing moves storage and compute resources out of the central data center and closer to the source of data.”
With edge computing, data is processed and analyzed at the edge, and only the results of the computing work are sent back to the main data center for further review and interaction. This approach minimizes the delay caused by transmitting large amounts of raw data to a centralized data center, enabling businesses to derive real-time business insights and make data-driven decisions with greater efficiency.
Real-Time Business Insights
Edge computing enables businesses to access real-time business insights by processing and analyzing data at the edge. This means that organizations can obtain immediate feedback and make timely decisions based on up-to-date information. Real-time business insights empower businesses to respond swiftly to changing market conditions, identify emerging trends, or address potential issues before they escalate.
Equipment Maintenance Predictions
Edge computing architecture also facilitates equipment maintenance predictions. By processing data at the edge, businesses can implement predictive analytics algorithms to detect signs of equipment malfunction or deterioration in real-time. These predictions enable proactive maintenance, preventing costly breakdowns and optimizing equipment performance and operational efficiency.
With edge computing, businesses can unlock the full potential of their data by leveraging real-time analytics and predictions for improved decision-making and operational efficiency. By moving storage and compute resources closer to the data source, edge computing architecture provides businesses with the agility and speed they need to thrive in today’s data-driven landscape.
How Does Edge Computing Work?
Edge computing revolutionizes the way data is processed and analyzed by deploying storage and compute resources at the location where data is produced. Unlike traditional enterprise computing, where data is transmitted across a Wide Area Network (WAN) and through the corporate Local Area Network (LAN), edge computing brings storage and compute resources closer to the data source, at the logical edge of the infrastructure.
This location-based computing approach offers several advantages, including minimizing latency and overcoming bandwidth limitations. By removing the need to transmit data to a centralized data center for processing and analysis, edge computing enables faster real-time decision-making, critical in time-sensitive situations.
With edge computing, the data center gets closer to the data, reducing the time it takes to process and analyze large amounts of data. This proximity eliminates the delays caused by data transmission across WAN and corporate LAN, resulting in reduced latency and improved performance.
Bandwidth limitations are also addressed by edge computing. By processing and analyzing data at the edge, businesses can minimize the amount of data that needs to be transmitted back to the central data center. This reduces network congestion and ensures efficient data transfer, even with limited bandwidth.
Overall, edge computing simplifies data processing and analysis by bringing the necessary resources closer to the data source. By minimizing latency and overcoming bandwidth limitations, it enables faster real-time decision-making and improves the efficiency of data-driven operations.
“Edge computing brings storage and compute resources closer to the data source, addressing the challenges posed by latency and bandwidth limitations.”
Edge Computing Workflow:
- Data is produced at the source, such as IoT devices or sensors.
- Storage and compute resources are deployed at the edge, in close proximity to the data source.
- Data is processed and analyzed locally, eliminating the need for transmission to a central data center.
- Only the results of the analysis are sent back to the central data center for further review and interaction.
By adopting this decentralized approach, edge computing enables businesses to harness real-time insights and make critical decisions without the delays caused by data transmission and network limitations.
Traditional Computing | Edge Computing |
---|---|
Data is generated at the source. | Data is generated at the source. |
Data is transmitted across a WAN to a central data center. | Data is processed and analyzed at the location where it is produced. |
Data processing and analysis occur at a central data center. | Data processing and analysis occur at the edge, closer to the data source. |
Results are sent back to the client endpoint. | Results are sent back to the central data center for further review and interaction. |
Edge Computing for AI
Edge computing and AI integration revolutionize data processing by bringing AI capabilities closer to the data source. With edge AI, AI algorithms and models are directly deployed on local edge devices like sensors or IoT devices. This allows for faster processing speeds and real-time issue detection by running AI algorithms on the edge devices themselves. By leveraging edge computing architecture, such as Multi-Access Edge Computing (MEC), the IoT, AI, and 5G networks seamlessly integrate to enable coherent and efficient processing of massive amounts of data generated by IoT devices across various industries.
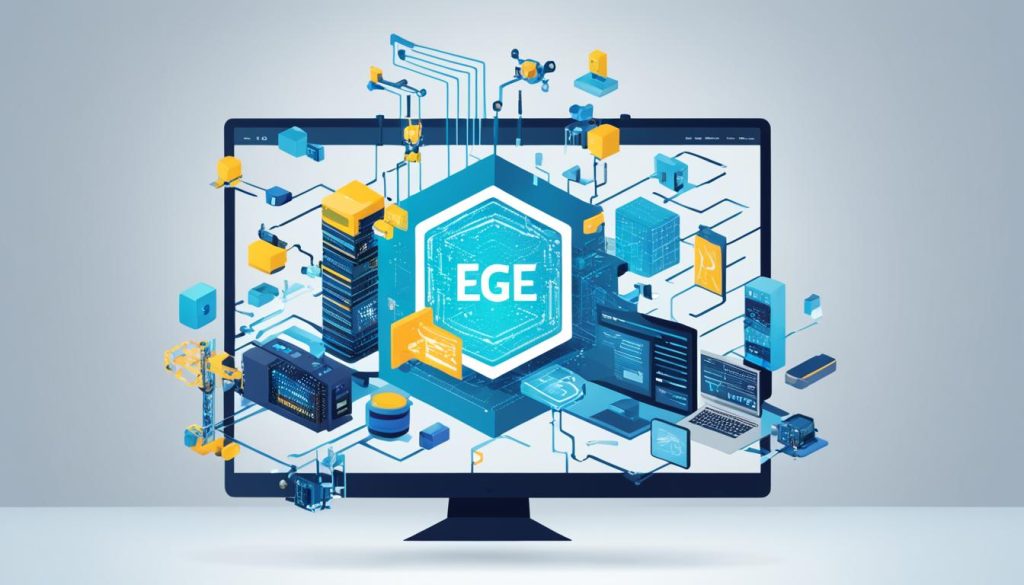
Edge computing for AI has far-reaching applications in industries such as healthcare, manufacturing, autonomous vehicles, and smart cities. In healthcare, edge computing enables real-time data processing and analysis, facilitating faster diagnosis, personalized treatment plans, and remote patient monitoring. In manufacturing, edge AI enables predictive maintenance, reducing downtime and optimizing production efficiency. Autonomous vehicles benefit from edge computing by enabling real-time decision-making based on data from various sensors and sources. Smart cities leverage edge computing to improve public safety, optimize energy usage, and enhance infrastructure management.
By bringing AI capabilities to edge devices, edge computing empowers businesses and organizations with real-time data processing and analysis, enabling them to extract valuable insights and make informed decisions without relying solely on distant data centers. The integration of edge computing and AI is driving the next wave of innovation and efficiency in data processing, ushering in a future where real-time insights and intelligent decision-making are the new normal.
Edge Computing Benefits and Use Cases
Edge computing offers a wide range of benefits to industries, empowering real-time decision-making with reduced latency. By bringing data processing capabilities closer to the source, edge computing enables immediate adjustments and optimizations in various sectors, including manufacturing, transportation, and smart cities.
One of the key advantages of edge computing is its ability to enable real-time monitoring and analysis in industrial automation. Edge devices equipped with AI algorithms can continuously monitor production lines, detect anomalies in real-time, and facilitate predictive maintenance. This proactive approach enhances operational efficiency, minimizes downtime, and improves overall productivity.
Moreover, in the transportation sector, edge computing plays a critical role in route optimization. By analyzing real-time data from various sources, including sensors and IoT devices, edge computing enables intelligent route planning, leading to reduced travel time, fuel consumption, and carbon emissions. This has significant implications for logistics companies, public transportation systems, and delivery services.
Smart cities also benefit from edge computing by enabling efficient urban management and resource allocation. With real-time data processing capabilities, edge devices and edge computing infrastructure can dynamically respond to changing conditions, optimizing energy consumption, traffic flow, and waste management. This ensures sustainable and effective city management.
Edge computing use cases extend beyond industrial automation and smart cities. In healthcare, edge computing enables real-time patient monitoring, remote consultations, and efficient healthcare delivery. In the field of autonomous vehicles, edge computing plays a crucial role in processing the vast amounts of data generated by sensors, ensuring real-time decision-making for safe and reliable navigation.
By integrating edge computing with AI algorithms, industries can unlock countless innovative use cases. From real-time data processing to intelligent decision-making, the combination of edge computing and AI revolutionizes industries and unlocks insights that were previously unattainable.
Overall, edge computing brings about transformative benefits and use cases across various sectors, enabling reduced latency, real-time decision-making, and enhanced efficiency.
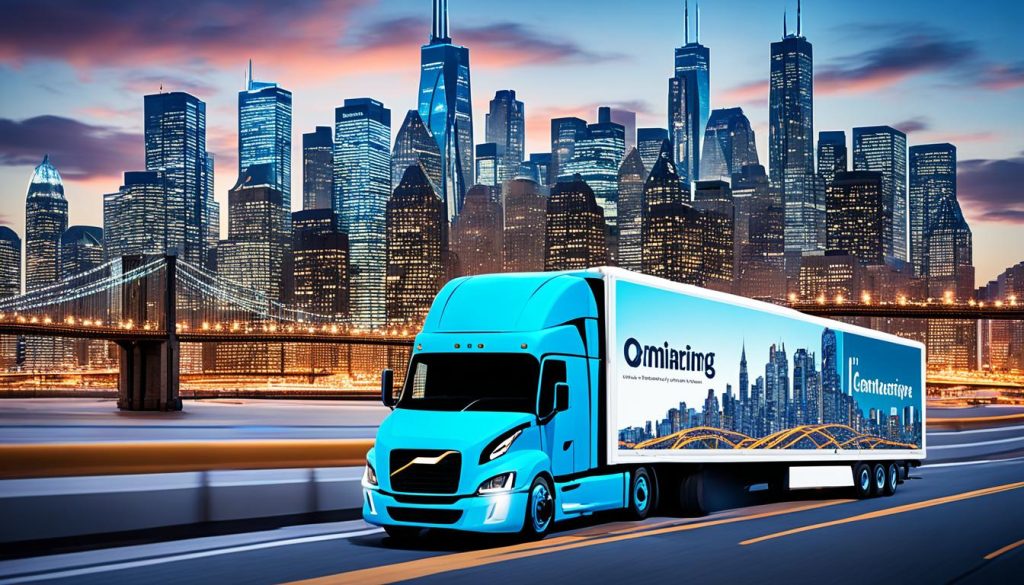
Industry | Use Case |
---|---|
Manufacturing | Real-time anomaly detection in production lines |
Transportation | Intelligent route optimization |
Smart Cities | Dynamic urban resource management |
Healthcare | Real-time patient monitoring and remote consultations |
Autonomous Vehicles | Real-time data processing for safe navigation |
Edge Computing vs. Cloud and Fog Computing
When it comes to distributed computing architectures, edge computing, cloud computing, and fog computing are three closely related concepts. While they share similarities, each approach has its own unique characteristics and applications. Let’s explore the differences between these three computing paradigms.
Edge Computing
Edge computing is a decentralized approach that brings storage and compute resources to the location where data is produced. Unlike cloud computing, which relies on centralized data centers, edge computing processes data closer to the data source, minimizing latency and bandwidth limitations. This proximity enables faster real-time decision-making and supports use cases such as industrial automation, smart cities, and predictive maintenance.
Cloud Computing
Cloud computing, on the other hand, focuses on the centralization of data processing and storage. In a cloud computing architecture, data is transmitted to remote data centers for analysis and computation. This centralized approach allows for scalability and accessibility but may introduce challenges related to latency and dependency on an internet connection. Cloud computing is widely used in various industries for tasks such as data storage, software development, and virtualization.
Fog Computing
Fog computing operates within the scope of a specific environment, utilizing a series of fog node deployments. These fog nodes are responsible for collecting, processing, and analyzing data generated within their vicinity. Fog computing serves as an intermediate layer between edge and cloud computing, enabling localized data processing while still leveraging the resources available in the cloud. This approach is particularly useful in scenarios where real-time data analysis and response are critical, such as in autonomous vehicles or smart infrastructure.
Edge computing deploys storage and compute resources at the location where data is produced, while cloud computing relies on centralized data centers that are often remote from the data source. Fog computing operates within the scope of an environment, utilizing a series of fog node deployments to collect, process, and analyze data.
While fog computing and edge computing share similar architectures, it’s important to note that they are not interchangeable terms. Each approach serves different purposes and presents unique advantages depending on the specific requirements of the use case.
Conclusion
Edge computing is revolutionizing data processing by bringing storage and compute resources closer to the data source. With its ability to enable real-time data processing, edge computing improves efficiency and enhances decision-making. By integrating AI with edge computing, organizations can harness valuable insights and intelligence without relying on distant data centers. This combination of edge computing and AI has vast applications across diverse industries, including healthcare, manufacturing, autonomous vehicles, and smart cities.
As technology continues to advance, edge computing will play a vital role in shaping the future of data centers and AI-driven innovations. Its real-time data processing capabilities and efficiency enhancements make it a compelling solution for organizations seeking to optimize processes and make data-driven decisions more effectively. Edge computing empowers businesses to analyze and act upon vast volumes of data where it is generated, paving the way for faster, smarter, and more agile operations.
From reducing latency and enabling real-time decision-making to improving overall productivity and automating processes, edge computing offers tangible benefits to businesses of all sizes. It allows organizations to unlock the full potential of their data, empowering them to gain a competitive edge in the digital era. As edge computing technologies continue to mature and evolve, we can expect to see even more exciting use cases and transformative applications across various industries.
FAQ
What is edge computing?
Edge computing is a distributed IT architecture that processes client data at the periphery of the network, closer to the data source. It enables businesses to handle the virtual flood of data generated by sensors and IoT devices in real-time, without the limitations of traditional centralized data centers.
How does edge computing work?
Edge computing moves storage and compute resources out of the central data center and closer to the source of data. It eliminates the need to transmit raw data to a central data center for processing and analysis. Data is processed at the edge, where it is generated, allowing for faster data processing and analysis.
What is the benefit of integrating AI with edge computing?
Edge computing and AI integration revolutionize data processing by bringing AI capabilities closer to the data source. This enables faster processing speeds and real-time issue detection by running AI algorithms on locally deployed edge devices.
What are the benefits and use cases of edge computing?
Edge computing brings numerous benefits to industries and enables real-time decision-making with reduced latency. It allows for immediate adjustments and optimizations in industries such as manufacturing, transportation, and smart cities. Edge devices with AI algorithms can continuously monitor production lines, detect anomalies in real-time, optimize routes, and perform predictive maintenance.
What is the difference between edge computing, cloud computing, and fog computing?
Edge computing deploys storage and compute resources at the location where data is produced, while cloud computing relies on centralized data centers. Fog computing operates within the scope of an environment, utilizing a series of fog node deployments to collect, process, and analyze data.
How does edge computing transform data processing?
Edge computing brings storage and compute resources closer to the data source, enabling real-time data processing and improved efficiency in decision-making. The integration of AI with edge computing enhances the capabilities of data processing, enabling organizations to harness insights and intelligence without relying on distant data centers.