AI accelerators, such as those used in neural networks, generate a significant amount of heat that needs to be effectively cooled to ensure peak performance. Data centers and edge devices that house AI accelerators require efficient cooling techniques to prevent overheating and maintain optimal functionality. In recent years, there have been significant advancements in cooling technology to address these challenges and improve energy efficiency in AI accelerators.
Key Takeaways:
- Cooling techniques are crucial for maintaining the performance and longevity of AI accelerators in neural networks
- Efficient cooling ensures optimal functionality and prevents overheating
- Advancements in cooling technology have led to improved energy efficiency in AI accelerators
- Implementing effective cooling techniques reduces energy consumption and minimizes environmental impact
- Investing in cooling optimization unlocks the full potential of AI accelerators
The Importance of Cooling in Data Centers and Edge Devices
In the world of AI accelerators, cooling plays a crucial role in maintaining performance and maximizing the lifespan of these powerful devices. Whether housed in data centers or edge devices, AI accelerators generate a considerable amount of heat during operation. Without effective cooling, the consequences can be detrimental – reduced performance, increased energy consumption, and potential hardware damage.
Implementing efficient cooling techniques is therefore essential to ensure optimal operation and safeguard AI accelerators in data centers and edge devices. By regulating temperature levels, cooling systems help prevent overheating, ensuring consistent and reliable performance. This not only maintains the computational efficiency of the AI accelerators but also improves energy utilization, leading to cost savings.
When it comes to data centers, the need for effective cooling is amplified. These facilities host a multitude of AI accelerators, creating a concentrated heat source. Without proper temperature management, the accumulated heat can have severe consequences, such as thermal throttling or even complete system failure.
“Effective cooling is imperative to guarantee the maximum potential of AI accelerators in data center environments.”
Similarly, edge devices, which often have limited space for cooling infrastructure, require innovative cooling solutions to maintain optimal conditions. The significance of efficient cooling becomes even more pronounced in remote or harsh environments, where maintaining AI accelerator performance is critical.
The Impact of Inadequate Cooling
Insufficient cooling measures can result in several detrimental effects on AI accelerators and the overall system:
- Reduced Performance: Excessive heat can lead to thermal instability and cause AI accelerators to operate at lower frequencies, reducing their overall performance.
- Increased Energy Consumption: When AI accelerators run hot, they require more power to maintain stability. This leads to increased energy consumption and higher operating costs.
- Hardware Damage: Prolonged exposure to high temperatures can cause irreversible damage to AI accelerators, reducing their lifespan and necessitating costly replacements.
The Role of Efficient Cooling Techniques
Implementing efficient cooling techniques is vital to counteract the challenges posed by the heat generated by AI accelerators. Advanced cooling solutions, such as liquid cooling and immersive cooling, provide improved heat dissipation and superior temperature control.
Liquid cooling involves circulating coolant directly through the AI accelerator components, effectively drawing out the excess heat. This method maximizes cooling capacity and enables more efficient heat transfer, improving the overall performance and longevity of the devices.
On the other hand, immersive cooling submerges AI accelerators in a specialized liquid, which absorbs heat directly from the components. The liquid conducts heat away from the device, keeping the temperatures within an optimal range.
Cooling systems, tailored to the unique requirements of AI accelerators, can significantly enhance their performance, reduce energy consumption, and prolong their lifespan. By utilizing cutting-edge cooling technologies and implementing well-designed cooling infrastructures, organizations can unlock the full potential of their AI accelerators in data centers and edge devices alike.
Current Cooling Solutions for AI Accelerators
As the demand for AI accelerators continues to grow, the need for efficient cooling solutions becomes increasingly important. Various cooling methods have been developed to address the unique cooling requirements of AI accelerators while prioritizing energy efficiency. These solutions range from traditional techniques, such as pumps, chillers, and cooling towers, to more advanced methods like liquid cooling and immersive cooling.
**Liquid cooling** is a cutting-edge approach that involves circulating specialized coolant directly through the hardware components of AI accelerators. By directly targeting the heat-generating components, liquid cooling effectively dissipates heat and allows for precise temperature control. This technique enhances the energy efficiency of AI accelerators by reducing the strain on the cooling system and enabling more effective heat removal.
On the other hand, **immersive cooling** takes cooling to a new level by submerging AI accelerators in a specialized liquid. This liquid has excellent heat transfer properties, ensuring efficient heat dissipation from the AI accelerators. Immersive cooling not only improves energy efficiency but also provides effective temperature management, minimizing the risk of overheating and maximizing performance.
Both liquid cooling and immersive cooling solutions aim to improve energy efficiency and ensure optimal operation of AI accelerators. By effectively managing heat generation and dissipation, these cooling solutions contribute to the longevity and performance of AI accelerators.
Advancements in Machine Learning for Cooling Optimization
Machine learning techniques are playing a crucial role in optimizing the cooling of AI accelerators in data centers. By leveraging the power of machine learning algorithms, specific to cooling optimization, significant advancements have been made in improving energy efficiency and performance.
One key application of machine learning in cooling optimization is the training of neural networks on large datasets collected from sensors within the data center. This process allows the machine learning algorithms to gain a comprehensive understanding of the complex dynamics and thermal characteristics of the data center environment.
With this understanding, the machine learning algorithms can then accurately predict and optimize cooling efficiency, enabling AI accelerators to operate at their full potential while minimizing energy consumption. The results have been impressive, with observed energy savings of up to 40% in cooling energy consumption.
Through the analysis of real-time data and the continuous learning capabilities of machine learning algorithms, cooling optimization can be dynamically adjusted based on the specific requirements of the AI accelerators and the data center environment. This adaptability ensures that cooling solutions are tailored to the unique demands of AI workloads, further enhancing their efficiency.
This synergy between machine learning and cooling optimization holds great promise for the future of AI accelerators. As advancements in machine learning continue, we can expect even more sophisticated algorithms and techniques to be developed, further improving the overall energy efficiency and performance of AI accelerators.
Benefits of Machine Learning for Cooling Optimization
The integration of machine learning technology into cooling optimization for AI accelerators brings several benefits:
- Enhanced Energy Efficiency: Machine learning algorithms can fine-tune cooling systems in real-time, significantly reducing energy consumption and operating costs.
- Improved Performance: By optimizing cooling efficiency, AI accelerators can maintain optimal operating temperatures, ensuring consistent peak performance.
- Reduced Environmental Impact: Energy savings achieved through machine learning-based cooling optimization contribute to a greener, more sustainable approach to AI computing.
Overall, the advancements in machine learning for cooling optimization have proven to be a game-changer for optimizing the performance and energy efficiency of AI accelerators. As the AI industry continues to grow, the integration of machine learning technology will become even more vital in ensuring the success and sustainability of AI-powered applications and data centers.
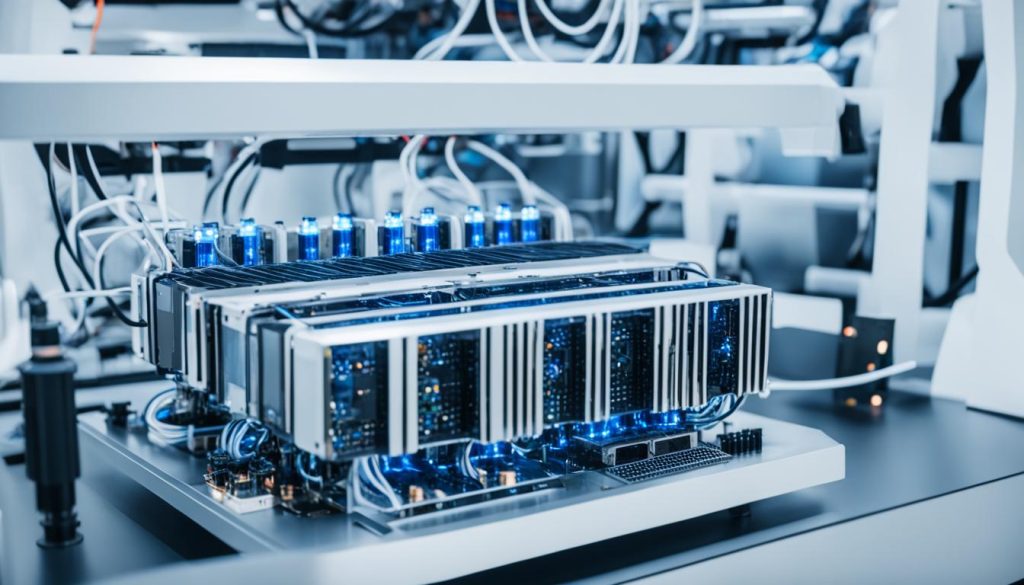
The Benefits of Effective Cooling for AI Accelerators
Implementing effective cooling techniques for AI accelerators offers several benefits. Firstly, it ensures the optimal performance of the accelerators by preventing overheating and maintaining stable operating temperatures. This leads to improved computational efficiency and reduced energy consumption. Secondly, efficient cooling extends the lifespan of AI accelerators, reducing the need for frequent replacements and minimizing downtime. Lastly, effective cooling techniques contribute to environmental sustainability by reducing energy consumption and greenhouse gas emissions. Overall, investing in cooling optimization for AI accelerators offers tangible benefits in terms of performance, longevity, and environmental impact.
When AI accelerators operate at high temperatures, their performance can be significantly compromised. Effective cooling techniques play a crucial role in maintaining the accelerators’ temperatures within the recommended operating range, ensuring consistent and reliable performance.
By effectively cooling AI accelerators, organizations can achieve improved computational efficiency. Cooler accelerators can process data more efficiently, resulting in faster processing times, quicker results, and enhanced productivity.
In addition to improving performance, efficient cooling extends the lifespan of AI accelerators. By preventing overheating and minimizing thermal cycling, which can cause wear and tear on components, effective cooling techniques reduce the need for frequent replacements, thereby lowering maintenance costs and minimizing downtime.
Investing in cooling optimization for AI accelerators also aligns with environmental sustainability goals. By reducing energy consumption, organizations can contribute to a greener future and minimize their carbon footprint. Additionally, efficient cooling techniques contribute to a more eco-friendly data center or edge device by reducing the release of greenhouse gases into the atmosphere.
To summarize, the benefits of effective cooling for AI accelerators include:
- Optimal performance and improved computational efficiency
- Extended lifespan and reduced maintenance costs
- Contribution to environmental sustainability and reduced energy consumption
Case Study:
Company XYZ, a leading provider of AI solutions, implemented an innovative cooling system for their data center’s AI accelerators. By utilizing liquid cooling technology, they were able to maintain optimal temperatures and achieve a 30% reduction in energy consumption. This led to improved performance, longer accelerator lifespan, and significant cost savings. The environmentally-friendly system also aligned with Company XYZ’s commitment to sustainability.
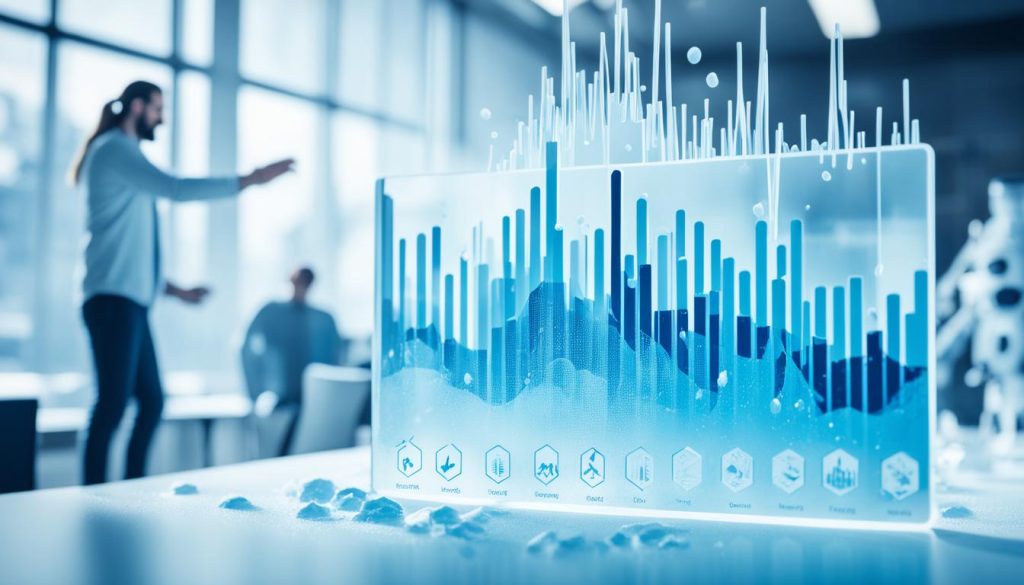
Benefits of Effective Cooling for AI Accelerators | |
---|---|
Optimal Performance | Prevents overheating and maintains stable operating temperatures for consistent and reliable performance. |
Improved Computational Efficiency | Cooler accelerators process data more efficiently, resulting in faster processing times and enhanced productivity. |
Extended Lifespan | Reduces wear and tear on components, minimizing the need for frequent replacements and lowering maintenance costs. |
Environmental Sustainability | Reduces energy consumption and greenhouse gas emissions, contributing to a more eco-friendly data center or edge device. |
Conclusion
Effective cooling techniques are essential for optimizing the performance and longevity of AI accelerators in neural networks. As the demand for computing power continues to rise in data centers and edge devices, it becomes increasingly crucial to prioritize cooling efficiency to reduce energy consumption, improve computational efficiency, and minimize environmental impact.
Advancements in machine learning have significantly contributed to cooling optimization, resulting in notable energy savings. By leveraging machine learning algorithms and analyzing data collected from sensors within the data center, organizations can gain insights into the complex dynamics of the environment and make informed predictions to enhance cooling efficiency.
Investing in innovative and efficient cooling solutions unlocks the full potential of AI accelerators and allows organizations to make substantial contributions to a more sustainable future. By implementing effective cooling techniques, companies can achieve optimal performance, extend the lifespan of AI accelerators, and minimize the need for frequent replacements. Additionally, these techniques play a vital role in reducing energy consumption and greenhouse gas emissions, contributing to environmental sustainability.
FAQ
What are AI accelerators?
AI accelerators are specialized hardware devices that are designed to accelerate the performance of artificial intelligence (AI) computations. They are commonly used in neural networks to process and analyze large amounts of data at high speeds.
Why is cooling important for AI accelerators in data centers and edge devices?
Cooling is important for AI accelerators in data centers and edge devices because these devices generate a significant amount of heat during operation. Without effective cooling, the excessive heat can lead to reduced performance, increased energy consumption, and potential damage to the hardware.
What are the different cooling solutions available for AI accelerators?
There are various cooling solutions available for AI accelerators, including traditional methods such as pumps, chillers, and cooling towers. More advanced techniques such as liquid cooling, which involves circulating coolant directly through the hardware components, and immersive cooling, which submerges the accelerators in a specialized liquid, are also used.
How does machine learning optimize cooling for AI accelerators?
Machine learning optimizes cooling for AI accelerators by training neural networks on large datasets collected from sensors within the data center environment. This allows machine learning algorithms to understand the complex dynamics of the environment and make predictions to optimize cooling efficiency, resulting in significant energy savings.
What are the benefits of effective cooling for AI accelerators?
Effective cooling for AI accelerators offers several benefits. It ensures optimal performance by preventing overheating and maintaining stable operating temperatures, improves computational efficiency, extends the lifespan of accelerators, reduces the need for frequent replacements, minimizes downtime, and contributes to environmental sustainability by reducing energy consumption and greenhouse gas emissions.