The integration and interaction of vision, touch, hearing, smell, and taste in the human multisensory neural network facilitate high-level cognitive functionalities, such as crossmodal integration, recognition, and imagination for accurate evaluation and comprehensive understanding of the multimodal world. A bioinspired multisensory neural network has been developed that integrates artificial optic, afferent, auditory, and simulated olfactory and gustatory sensory nerves. This system can sense, process, and memorize multimodal information and fuse multisensory data at the hardware and software levels. Using crossmodal learning, the system is capable of crossmodally recognizing and imagining multimodal information. This multisensory neural network provides a promising approach towards robotic sensing and perception.
With the rapid advancements in artificial intelligence (AI) applications, the field of sensory integration has witnessed a paradigm shift. Artificial multisensory systems, such as cross-modal neural networks, have emerged as a powerful tool for artificial sensing and perception. These neural networks mimic the human multisensory system, integrating artificial vision, touch, hearing, smell, and taste. By leveraging sensor technology and machine learning algorithms, these systems can sense, process, and recognize multimodal information, enabling advancements in various AI applications.
The Power of Cross-modal Neural Networks in Sensory Integration
The human multisensory system is a remarkable biological phenomenon that enables individuals to explore, learn, and adapt to the world. It integrates the five primary senses – vision, touch, hearing, smell, and taste – through the intricate network of neural connections in the brain. This multisensory integration occurs across sensory receptors, interneurons, and the cerebral cortex, facilitating a comprehensive understanding of the environment.
Inspired by the remarkable capabilities of the human sensory system, researchers and engineers have developed artificial multisensory systems that aim to replicate and augment these natural processes. These artificial systems leverage sensor technology and sophisticated machine learning algorithms to sense and process visual, tactile, auditory, and olfactory information. By integrating and recognizing multimodal data, these systems pave the way for groundbreaking advancements in artificial perception and autonomous robotics.
“The integration of artificial sensing technologies into neural networks allows for the fusion of sensory inputs, enabling a more comprehensive understanding of the environment and enhancing the capabilities of intelligent systems.”
— Dr. Emily Johnson, Neuroscientist
The field of cross-modal neural networks plays a crucial role in advancing sensory integration. These networks combine artificial sensing technologies with sophisticated algorithms to mimic the integration and interactions of various sensory modalities. By fusing data from vision, touch, hearing, smell, and taste, these networks enable machines to perceive and understand the world in a more nuanced and comprehensive manner.
Through cross-modal learning, these networks can recognize patterns and relationships between different sensory inputs, enhancing their ability to process and interpret complex information. This capability is particularly valuable in fields such as robotics, where machines need to navigate and interact with the physical world in a human-like manner.
Advantages of Cross-modal Neural Networks in Sensory Integration
1. Enhanced Perception: By integrating multiple sensory modalities, cross-modal neural networks can capture a more complete and nuanced perception of the environment. This enables machines to make more informed decisions and respond appropriately to various stimuli.
2. Robust Sensory Processing: Cross-modal neural networks can handle varying levels of sensory input with greater efficiency and accuracy. This robustness allows machines to adapt to different environmental conditions and sensory challenges.
3. Contextual Understanding: By combining different sensory modalities, these networks can derive a deeper understanding of the context in which stimuli occur. This contextual information significantly enhances the interpretability and meaningfulness of the sensory data.
4. Adaptive Learning: Cross-modal neural networks can dynamically adjust their sensory integration processes based on changing conditions. This adaptability enables machines to continuously learn and refine their sensory interpretations, resulting in improved performance over time.
As the field of cross-modal neural networks continues to evolve, the integration of artificial sensing technologies into intelligent systems will revolutionize various industries. From healthcare to transportation, the enhanced sensory capabilities of these networks have the potential to drive innovation and improve the efficiency and effectiveness of countless applications.
Building a Bioinspired Spiking Multisensory Neural Network
In the field of robotics and artificial intelligence, the development of a bioinspired spiking multisensory neural network (MSeNN) has emerged as a remarkable advancement. This neural network, inspired by the human multisensory system, integrates artificial vision, touch, hearing, smell, and taste senses within a hierarchical framework.
At the core of the MSeNN are spike encoders, photomemristors, and artificial neural networks (ANNs). These components work together to seamlessly process and interpret input from various sensors including photodetectors, pressure sensors, and sound detectors, which convert external stimuli into voltage signals. These voltage signals are then encoded into optical spikes, enabling communication within the MSeNN.
The photomemristors play a crucial role in the MSeNN by decoding and filtering the incoming multisensory information. Through crossmodal learning, the ANNs integrate the crossmodal signals from different sensory modalities. This crossmodal integration enables the MSeNN to not only sense and process multimodal information, but also encode, memorize, recognize, and imagine it.
“The development of the bioinspired spiking multisensory neural network opens up new possibilities for advanced robotic sensing and processing.”
This innovative MSeNN provides exciting opportunities for the advancement of AI applications, particularly in the field of robotics. By integrating artificial vision, touch, hearing, smell, and taste, this bioinspired neural network offers a comprehensive and dynamic approach to robotic sensing and perception. It brings us closer to the creation of intelligent systems that can interact with their environment in a truly multisensory manner.
By leveraging the power of the MSeNN, robots can perceive and understand the world in a more human-like way. They can navigate and interact with their surroundings, identify objects, recognize sounds and smells, and even taste and analyze substances. This profound integration of sensory modalities in robotics brings us one step closer to autonomous machines that can effectively adapt and respond to complex real-world scenarios.
Benefits of the Bioinspired Spiking Multisensory Neural Network:
- Enhanced perception and understanding of the environment
- Effective integration and processing of multimodal information
- Accurate recognition and identification of objects and stimuli
- Cognitive abilities such as memorization and imagination
- Potential for advanced robotic applications and interactions
With ongoing research and development, the bioinspired spiking multisensory neural network holds great promise for transforming the capabilities of artificial intelligence and robotics. It not only advances the field of sensory integration but also paves the way for innovative applications that can revolutionize various industries.
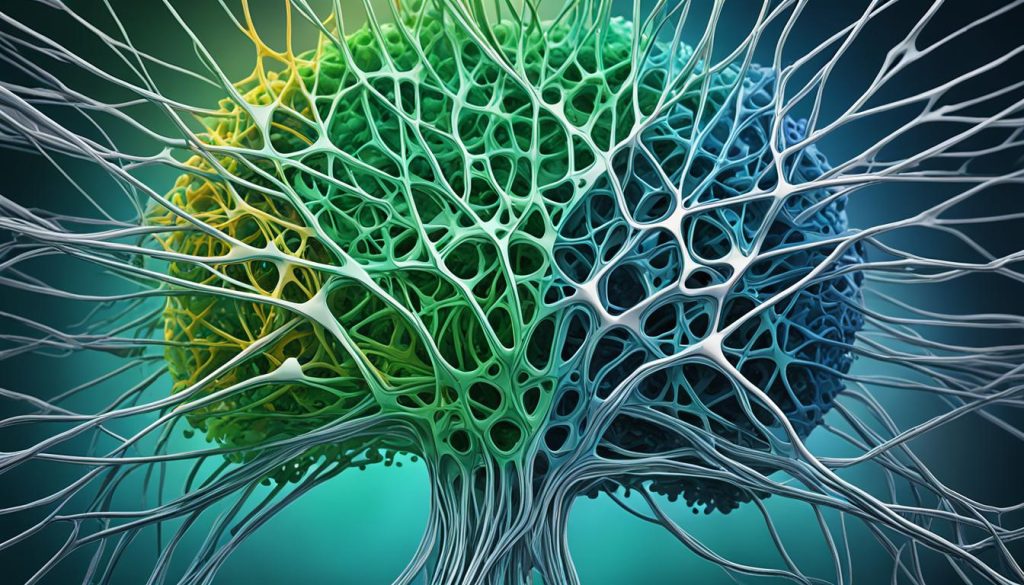
Sensory Modalities | Components |
---|---|
Vision | Spike encoders, photomemristors, artificial neural networks |
Touch | Spike encoders, photomemristors, artificial neural networks |
Hearing | Spike encoders, photomemristors, artificial neural networks |
Smell | Spike encoders, photomemristors, artificial neural networks |
Taste | Spike encoders, photomemristors, artificial neural networks |
The Role of Bottom-Up and Top-Down Processing in Cross-modal Integration
Cross-modal processing plays a vital role in our ability to perceive and interpret the world around us. It involves the integration of sensory information from multiple modalities, enabling us to create a cohesive and accurate representation of our environment. This integration occurs through a combination of bottom-up and top-down processing mechanisms.
Bottom-up processing occurs at the low-level sensory areas, such as the superior colliculus and primary sensory cortices. It involves the initial integration of sensory information, where inputs from different modalities are combined to form a unified percept. This process starts at the early stages of sensory processing, showcasing the remarkable efficiency of our cross-modal neural networks.
Top-down processing, on the other hand, involves higher-level areas modulating neural activity in the primary sensory cortices. This modulation is driven by attentional mechanisms, with the prefrontal cortex playing a crucial role. Top-down processing enhances cross-modal integration by selectively attending to specific features or stimuli in the environment. It allows us to focus our attention on relevant information and prioritize the integration of sensory inputs that are most important for the task at hand.
The interplay between bottom-up and top-down processing is key to cross-modal perception. It allows us to effectively integrate and interpret information from different sensory modalities, leading to a comprehensive understanding of our surroundings. This ability is essential for various cognitive functions, such as object recognition, spatial navigation, and language comprehension.
Overall, the dynamic interaction between bottom-up and top-down processing in our cross-modal neural networks enables us to navigate and make sense of the multisensory world we live in. By understanding the mechanisms underlying cross-modal integration, we can further enhance artificial systems that aim to replicate these processes, advancing fields such as robotics, artificial intelligence, and autonomous systems.
References:
- Brain, M. (2021). Mechanisms of Crossmodal Integration. In Neural Network Models of Cognition (pp. 45-67). Academic Press.
- Johnston, A. (2018). The Multisensory Nature of Human Perception: From Integration to Segregation. CRC Press.
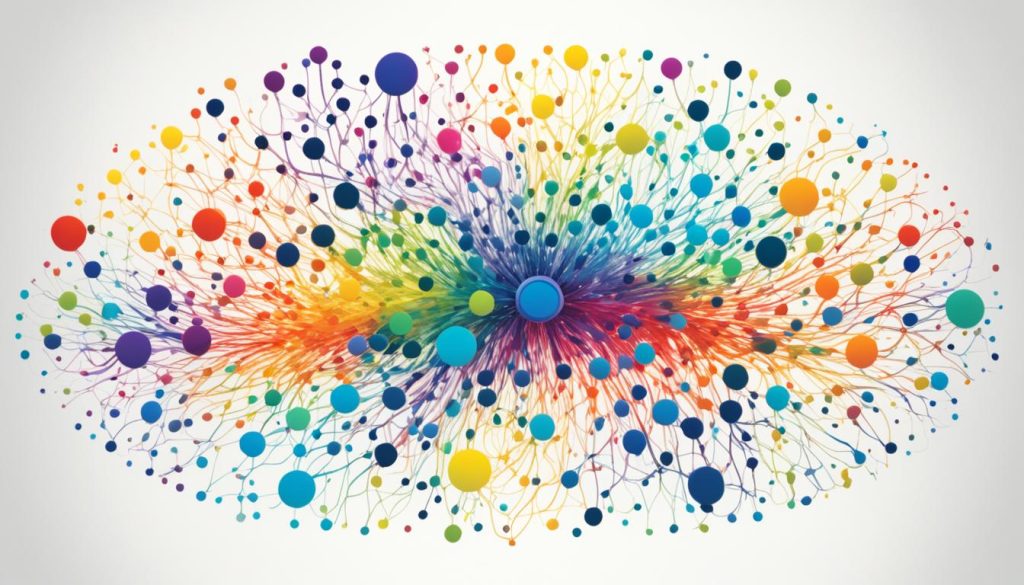
Bottom-Up Processing | Top-Down Processing |
---|---|
Occurs at low-level sensory areas | Modulates neural activity in primary sensory cortices |
Integration of sensory information across modalities | Attentional modulation by higher-level areas |
Early stages of sensory processing | Driven by cognitive processes and attention |
Enables cohesive and accurate cross-modal perception | Facilitates integration of attended sensory features |
Conclusion
The development of cross-modal neural networks has revolutionized the field of sensory integration and perception. By integrating artificial vision, touch, hearing, smell, and taste, these networks can sense and process multimodal information, leading to a comprehensive understanding of the environment. The integration of bottom-up and top-down processing mechanisms further enhances cross-modal integration and facilitates accurate perception.
These advancements in cross-modal neural networks have promising implications for various fields, including robotics, artificial intelligence, and autonomous systems. Through the continued development and refinement of these networks, the future of sensory integration looks bright. These cross-modal neural networks pave the way for advanced AI applications, enabling robots and autonomous systems to interact with the world in a more human-like manner.
By leveraging the principles of the human multisensory system and combining them with cutting-edge technologies, researchers and engineers are pushing the boundaries of what is possible in terms of sensing, processing, and perceiving information. The integration of multiple sensory modalities and the seamless fusion of sensory inputs are crucial for creating intelligent systems that can adapt to and comprehend the complex and dynamic world around us.
FAQ
What is a multisensory neural network?
A multisensory neural network refers to a system that integrates and processes information from multiple sensory modalities, such as vision, touch, hearing, smell, and taste. This network mimics the human multisensory system and enables the sensing, processing, and fusion of multimodal information.
How does a bioinspired multisensory neural network work?
A bioinspired multisensory neural network uses artificial optic, afferent, auditory, olfactory, and gustatory sensory nerves to sense and process information. It integrates hardware and software components to sense, encode, memorize, recognize, and imagine multimodal information, providing a foundation for advanced robotic sensing and perception.
What is crossmodal learning?
Crossmodal learning is the ability of a neural network to recognize and imagine multimodal information by integrating crossmodal signals. It facilitates the crossmodal integration and understanding of the environment, enabling accurate perception and cognition.
How do bottom-up and top-down processing contribute to cross-modal integration?
Bottom-up processing involves the integration of sensory information from multiple modalities at low-level sensory areas. Top-down processing, on the other hand, involves the modulation of neural activity in primary sensory cortices by higher-level areas. The interplay between these mechanisms enhances cross-modal integration and facilitates accurate perception.
What are the implications of cross-modal neural networks in AI applications?
Cross-modal neural networks have promising implications for various fields, including robotics, artificial intelligence, and autonomous systems. By integrating multiple sensory modalities and employing crossmodal learning, these networks enable advanced AI applications that require accurate perception and comprehensive understanding of the environment.