Custom AI chips are revolutionizing the field of artificial intelligence (AI) and machine learning, providing specialized hardware for optimized neural network functions. These chips are designed to meet the growing demand for high-performance hardware in industries such as healthcare, finance, and autonomous vehicles. With their ability to enhance performance and efficiency, custom AI chips have become an integral part of driving the AI revolution.
As machine learning algorithms require significant computational power, custom AI hardware is essential in meeting the requirements of AI tasks. While traditional graphics processing units (GPUs) have been widely used, custom AI chips offer several advantages. They deliver higher performance and efficiency, allowing companies to reduce costs and have greater control over their AI infrastructure.
When comparing custom AI chips to GPUs, there is a trade-off between performance and efficiency. While GPUs excel in raw performance with parallel processing capabilities, custom AI chips offer comparable performance while consuming less power. For example, Google’s Tensor Processing Units (TPUs) provide impressive performance with lower power consumption compared to traditional GPUs.
Leading tech companies such as Google, Meta, Amazon, Microsoft, and Tesla are actively developing their own custom AI chips. These chips power their machine learning infrastructure, accelerating AI workloads and driving advancements in AI technology. Moreover, other players like Tenstorrent, Apple, AMD, and Intel have also entered the custom AI chip market, highlighting its growing importance in shaping the future of AI hardware.
Key Takeaways:
- Custom AI chips are specialized hardware that optimize neural network functions in AI and machine learning.
- They provide higher performance and efficiency compared to traditional GPUs.
- Leading tech companies such as Google, Meta, Amazon, Microsoft, and Tesla are actively developing their own custom AI chips.
- Other players like Tenstorrent, Apple, AMD, and Intel have also entered the custom AI chip market.
- Custom AI chips have a significant impact on various AI applications across industries, pushing the boundaries of AI capabilities.
The Need for Custom AI Hardware in Machine Learning
Machine learning algorithms require significant computational power to process and analyze large amounts of data for pattern recognition and prediction. While traditional graphics processing units (GPUs) have been commonly used for AI tasks, custom AI hardware offers several advantages.
Custom AI hardware is specifically designed to meet the specific requirements of machine learning, providing higher performance and efficiency compared to GPUs. These specialized chips are optimized for the complex computations involved in training and running machine learning models, ensuring faster processing and more accurate results.
Developing custom AI hardware also allows companies to reduce costs and achieve greater control over their AI hardware infrastructure. By tailoring the hardware to their specific needs, organizations can optimize their machine learning workflows, reduce reliance on third-party solutions, and tailor their hardware to their unique applications and data requirements.
Advantages of Custom AI Hardware
“Custom AI hardware provides higher performance and efficiency compared to GPUs, enabling faster processing and more accurate results in machine learning.”
Some of the key advantages of custom AI hardware include:
- Optimized Performance: Custom AI hardware is designed specifically for the demands of machine learning, delivering superior performance compared to general-purpose GPUs. This results in faster training and inference times, allowing organizations to process larger datasets and derive insights quicker.
- Energy Efficiency: Custom AI chips are engineered to be power-efficient, ensuring that machine learning models can be trained and deployed without excessive energy consumption. This is especially crucial for large-scale machine learning applications, where minimizing power requirements can lead to significant cost savings.
- Scalability: Custom AI hardware can be scaled efficiently to handle increasing workloads. By designing hardware with scalability in mind, organizations can seamlessly upgrade their infrastructure to accommodate growing needs, future-proofing their machine learning capabilities.
Overall, the need for custom AI hardware arises from the increasing demand for high-performance and energy-efficient solutions in machine learning. As AI applications become more complex and data-intensive, specialized hardware is necessary to unlock the full potential of machine learning algorithms and drive advancements in various industries.
Comparison of Custom AI Hardware and GPUs | Performance | Efficiency |
---|---|---|
Custom AI Hardware | Higher performance for machine learning tasks | Optimized for energy efficiency |
GPUs | Higher raw performance for general-purpose computing | Less power-efficient for machine learning workloads |
Custom AI Chips vs. GPUs: A Comparison of Performance and Efficiency
When it comes to comparing custom AI chips and GPUs, there are trade-offs to consider in terms of performance and efficiency. GPUs have long been the go-to hardware for AI tasks, offering high raw performance with their parallel processing capabilities. However, custom AI chips excel in efficiency, delivering comparable performance while consuming less power.
Google’s Tensor Processing Units (TPUs) serve as a prime example of the evolution of custom AI chips. These specialized chips have undergone multiple generations of development, offering impressive performance with lower power consumption compared to traditional GPUs.
In terms of performance, GPUs have a clear advantage with their ability to handle intensive computational tasks in parallel. They are particularly well-suited for tasks that require massive amounts of floating-point calculations. However, this raw performance often comes at the expense of power consumption and energy efficiency.
“Custom AI chips provide a unique solution by optimizing hardware specifically for AI workloads. They bridge the gap between raw performance and power efficiency, offering comparable performance while consuming less power.”
Custom AI chips, on the other hand, are specifically designed to meet the requirements of machine learning and AI functions. These chips leverage specialized architectures and algorithms to maximize performance and minimize power consumption. This focus on efficiency makes them ideal for AI workloads where energy efficiency is a critical factor.
Custom AI Chips | GPUs |
---|---|
Optimized for AI workloads | General-purpose hardware |
Higher efficiency | Higher raw performance |
Lower power consumption | Higher power consumption |
As technology continues to advance, custom AI chips are expected to bridge the gap and become the hardware of choice for AI workloads. Their focus on efficiency and performance optimization will play a significant role in driving advancements in AI applications across industries.
Big Tech’s Custom AI Chip Developments Behind the Scenes
Leading tech companies such as Google, Meta (formerly Facebook), Amazon, Microsoft, and Tesla have been actively developing their own custom AI chips to power their machine learning infrastructure. These companies recognize the importance of specialized hardware in optimizing AI performance and efficiency.
One notable player in the field is Google, which has made significant advancements with its custom AI chip, the Tensor Processing Unit (TPU). TPUs are designed to accelerate machine learning workloads, providing faster processing and training times. Customers can also utilize TPUs through Google’s cloud platform, enabling accessibility for AI applications.
Meta has introduced its own custom AI chip named MTIA, focusing on efficiency and reducing reliance on traditional GPUs. With this chip, Meta aims to enhance the performance of its machine learning algorithms and pave the way for more efficient AI systems.
Similarly, Amazon has entered the custom AI chip market after acquiring Annapurna Labs. The company now offers instances powered by its custom AI chips named Inferential and Trainium. These chips provide improved performance and efficiency for AI workloads, further expanding Amazon’s cloud services capabilities.
Microsoft’s commitment to the development of custom AI chips is evident with the “Project Athena” initiative. Under this project, Microsoft aims to create its own AI chip to support its machine learning infrastructure, targeting enhanced performance and better control over AI hardware.
Tesla, known for its advancements in autonomous driving, has developed its own custom AI chip called Dojo D1. This chip is specifically designed to enhance the processing power of Tesla’s autonomous driving software, enabling more efficient and precise decision-making for self-driving vehicles.
The active involvement of these big tech companies in custom AI chip developments signifies the growing importance of specialized hardware in advancing AI technologies. These chips enable optimized performance, greater control over AI infrastructure, and pave the way for new possibilities in machine learning and beyond.
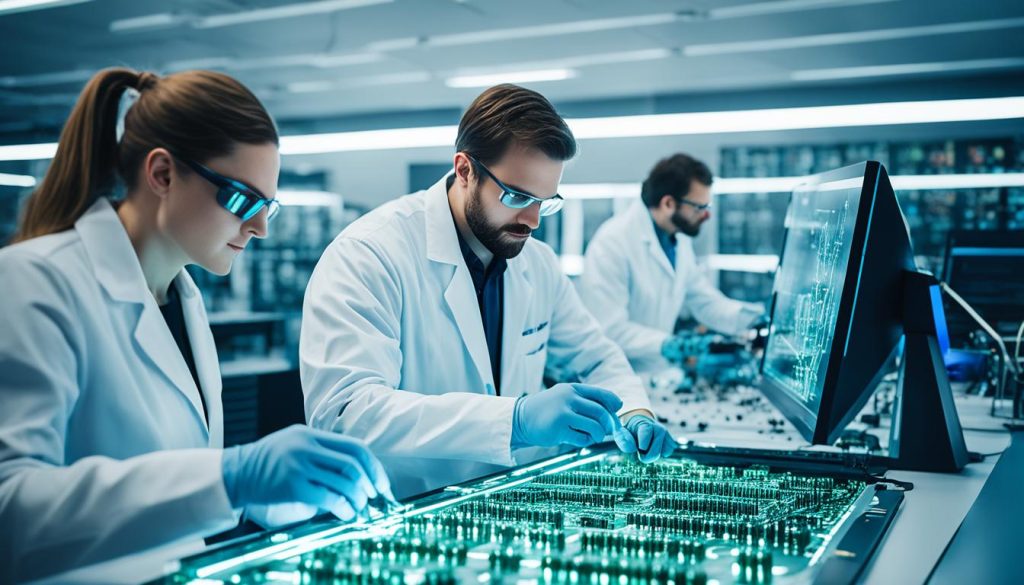
Company | Custom AI Chip |
---|---|
Tensor Processing Unit (TPU) | |
Meta (formerly Facebook) | MTIA |
Amazon | Inferential, Trainium |
Microsoft | Project Athena |
Tesla | Dojo D1 |
The Impact of Custom AI Hardware on AI Applications
Custom AI hardware plays a crucial role in driving advancements in various AI applications across multiple industries. By optimizing hardware specifically for AI workloads, developers can unlock a range of benefits that significantly impact the performance and efficiency of AI systems.
One key advantage of custom AI hardware is its ability to achieve higher performance levels compared to traditional hardware solutions. The specialized design of custom AI chips allows for efficient data processing and analysis, enabling faster training times and improved real-time decision-making capabilities.
Moreover, the development of custom AI hardware leads to enhanced energy efficiency. These chips are designed to minimize power consumption while maintaining optimal performance. As a result, organizations can not only reduce operational costs but also contribute to the sustainability of AI technologies.
Custom AI chips offer the potential to reduce inference costs and make AI models more accessible.
In addition to performance and efficiency benefits, custom AI hardware enables the development and training of more complex AI models. By leveraging the specialized capabilities of these chips, AI practitioners can push the boundaries of what AI systems can accomplish, opening up new possibilities for innovation.
Custom AI chips have made a significant impact on AI applications in various industries. In healthcare, they have contributed to the development of advanced diagnostic tools, personalized treatment plans, and drug discovery systems. In the autonomous vehicles sector, custom AI hardware has empowered vehicles with enhanced perception, decision-making, and control capabilities. Financial institutions have utilized custom AI chips to improve fraud detection, risk assessment, and algorithmic trading systems. The entertainment industry has also seen advancements in AI-driven content generation and immersive experiences.
Overall, the availability of custom AI hardware has accelerated the adoption and advancements of AI applications. Its optimization for AI workloads paves the way for improved performance, faster training, energy efficiency, and the development of more sophisticated AI models, all of which contribute to the growth and potential of AI in various industries.
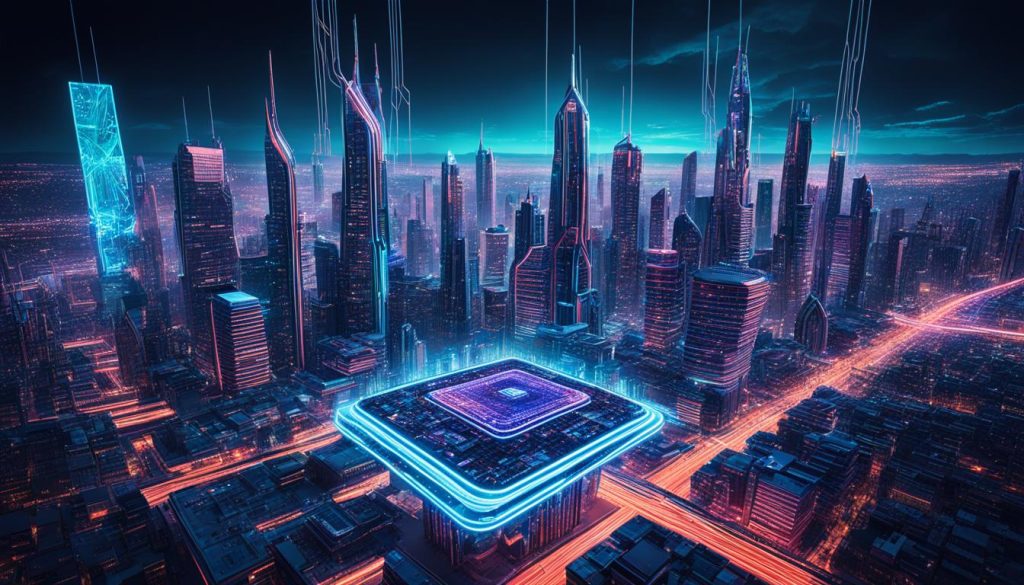
References:
- “Custom AI Hardware: Unlocking the Potential of AI Applications” – AI Tech Insights
- “The Impact of Custom AI Chips in Healthcare” – HealthTech Magazine
- “Enhancing Autonomous Vehicles with Custom AI Hardware” – Autonomous Driving News
- “Revolutionizing Financial Technology with Custom AI Chips” – FinTech Today
- “AI Applications in the Entertainment Industry: The Role of Custom AI Hardware” – Entertainment Tech Review
Other Players in the Custom AI Chip Market
In addition to the major tech companies mentioned earlier, there are several other players who have entered the custom AI chip market, further expanding the landscape and fostering competition. These companies are actively investing in the development of their own custom AI chips, recognizing the growing importance of specialized hardware in driving AI advancements and shaping the future of AI hardware.
Tenstorrent
Tenstorrent is a leading player in the custom AI chip market, specializing in high-performance AI solutions. Their custom AI chips are designed to deliver exceptional performance and efficiency, catering to the demanding needs of AI workloads. Tenstorrent’s focus on advanced architecture and software optimization sets them apart in this competitive market.
Apple
Apple, known for their innovation and consumer electronics, has also made significant strides in developing their own custom AI chips. By designing chips specifically tailored for their device ecosystem, Apple aims to enhance the on-device AI processing capabilities. This approach allows for faster and more efficient AI tasks, providing a seamless user experience across their product lineup.
AMD
AMD, a prominent player in the semiconductor industry, has ventured into the custom AI chip market with their high-performance computing solutions. Recognizing the demand for optimized AI hardware, AMD is leveraging their expertise in processor architectures to develop custom AI chips that deliver exceptional performance and scalability.
Intel
Intel, known for their dominance in the CPU market, is also expanding their presence in the custom AI chip market. With their significant manufacturing capabilities and expertise in semiconductor technology, Intel aims to develop custom AI chips that can deliver cutting-edge performance and power efficiency. Their focus on providing integrated solutions for AI workloads exemplifies their commitment to becoming a key player in the AI hardware space.
The presence of these companies in the custom AI chip market reflects the dynamic nature of the AI industry and the increasing reliance on specialized hardware for AI tasks. Their investments and developments in custom AI chips contribute to driving AI advancements, enabling faster processing, improved efficiency, and pushing the boundaries of what AI can achieve.
Company | Main Focus |
---|---|
Tenstorrent | High-performance AI solutions |
Apple | Optimized AI processing for their device ecosystem |
AMD | High-performance computing for AI workloads |
Intel | Integrated AI solutions with a focus on performance and power efficiency |
Conclusion
Custom AI chips have emerged as a critical component in the AI revolution, offering specialized hardware for enhanced neural network functions. These chips provide higher performance and efficiency compared to traditional GPUs, driving advancements in AI applications across industries. Companies like Google, Meta, Amazon, Microsoft, and Tesla are investing heavily in the development of custom AI chips, indicating a bright future for AI hardware.
With AI technology evolving rapidly, custom AI chips are set to play a significant role in reshaping the AI landscape. These chips unlock new possibilities in machine learning and are poised to revolutionize various industries. By optimizing hardware specifically for AI workloads, developers can achieve higher performance, faster training times, and improved energy efficiency.
As the demand for AI continues to grow, the custom AI chip market is witnessing increasing competition. Alongside major tech companies, players like Tenstorrent, Apple, AMD, and Intel are also entering the market, further driving advancements in AI hardware. Custom AI chips are paving the way for the future of AI, pushing the boundaries of what is possible and enabling the development of more complex AI models.
FAQ
What are custom AI chips?
Custom AI chips are specialized hardware components designed to optimize the performance and efficiency of neural network functions in the field of artificial intelligence (AI) and machine learning.
How do custom AI chips benefit machine learning?
Custom AI chips provide higher performance and efficiency compared to traditional graphics processing units (GPUs), allowing developers to achieve faster training times, improved energy efficiency, and the ability to develop and train more complex AI models.
How do custom AI chips compare to GPUs?
While GPUs offer higher raw performance with their parallel processing capabilities, custom AI chips excel in terms of efficiency, delivering comparable performance while consuming less power.
Which major tech companies are developing their own custom AI chips?
Major tech companies such as Google, Meta, Amazon, Microsoft, and Tesla have been actively developing their own custom AI chips to power their machine learning infrastructure.
What is the impact of custom AI hardware on AI applications?
Custom AI hardware enables higher performance, faster training times, improved energy efficiency, and the development of more complex AI models. It has driven significant advancements in AI applications in various industries such as healthcare, autonomous vehicles, finance, and entertainment.
Are there other players in the custom AI chip market?
Yes, apart from major tech companies, other players such as Tenstorrent, Apple, AMD, and Intel have also entered the custom AI chip market, reflecting the increasing importance of custom AI chips in driving AI advancements.
What is the significance of custom AI chips in the AI revolution?
Custom AI chips have emerged as a critical component in the AI revolution, providing specialized hardware for enhanced neural network functions. They offer higher performance and efficiency compared to traditional GPUs, driving advancements in AI applications and shaping the future of AI hardware.