AI-optimized data centers have revolutionized the way we handle large-scale AI computing requirements. These cutting-edge facilities not only offer breakthrough performance but also address critical challenges in cooling and power efficiency. By adopting advanced cooling systems and leveraging AI-driven technologies, these data centers are able to reduce energy consumption, lower costs, and ensure the seamless growth of AI capabilities without being hindered by infrastructure limitations.
In this article, we will explore the importance of AI-optimized data center design and how it supports the evolving power needs of AI-driven workloads. We will also delve into the recent guide launched by Schneider Electric, a leader in energy management and automation, which provides valuable insights on the physical infrastructure challenges and essential considerations for AI-optimized data center design.
Key Takeaways:
- AI-optimized data centers address cooling and power efficiency challenges to enable breakthrough performance in AI applications.
- Schneider Electric has launched a comprehensive guide that offers valuable insights and guidance for designing AI-optimized data centers.
- Applying AI and machine learning to data center operations can optimize various aspects, including cooling systems, power usage, and overall efficiency.
- AI-driven data center design improves energy efficiency, reduces emissions, and contributes to addressing climate change.
- Optimizing data centers for AI workloads is crucial for meeting the challenges of the AI disruption and ensuring seamless growth and scalability.
The Importance of AI-Optimized Data Center Design
With the exponential growth in AI applications across sectors such as healthcare, finance, manufacturing, transportation, and entertainment, the demand for AI-optimized data centers has never been higher. As organizations embrace the potential of artificial intelligence, data centers must adapt to meet the evolving power needs of AI-driven workloads.
The projections for AI workloads are staggering. According to industry experts, AI workloads are expected to grow at a compound annual growth rate (CAGR) of 26-36% by 2028. This significant growth places immense pressure on data centers to address challenges in power, cooling systems, infrastructure limitations, racks, and software tools in order to ensure efficient and optimized operations.
“AI computing requirements, cooling systems, and infrastructure limitations are primary concerns for data center operators aiming to meet the demands of AI workloads.” – Industry Expert
The computing requirements of AI applications are immense, demanding increased processing power and storage capacity. Data centers need to provide the necessary infrastructure to accommodate these requirements while ensuring optimal performance and scalability.
Furthermore, cooling systems play a critical role in AI-optimized data center design. Highly advanced cooling systems are essential to prevent overheating and maintain the optimal operating temperature for AI hardware. Efficient cooling systems help to reduce energy consumption and lower costs, making them integral to the success of AI-driven workloads.
Data centers also face infrastructure limitations when it comes to the physical space available for expansion. With the rapid growth of AI workloads, data centers must find innovative ways to maximize their infrastructure while ensuring the highest level of performance and efficiency.
To achieve AI-optimized data center design, data center operators must invest in advanced technologies, such as liquid cooling solutions, that can handle the increased power requirements of AI workloads. They must also prioritize scalability and flexibility, allowing for seamless expansion as AI applications continue to evolve.
By addressing AI computing requirements, cooling systems, and infrastructure limitations, data centers can support the growth and potential of AI applications, unlocking breakthrough performance and revolutionizing various industries.
Schneider Electric Launches Guide for AI-Optimized Data Center Design
As organizations across various sectors embrace artificial intelligence (AI) to drive innovation and gain a competitive edge, addressing the physical infrastructure challenges becomes paramount. Schneider Electric, a renowned leader in energy management and automation, recognizes this need and has launched a comprehensive guide titled “The AI Disruption: Challenges and Guidance for Data Center Design.”
This guide serves as a valuable resource for organizations seeking to fully leverage AI within their data centers. It provides insights and acts as a blueprint for designing AI-optimized data centers that can meet the demands of AI computing requirements. With expertise in data center infrastructure, Schneider Electric offers practical guidance to overcome the challenges associated with this disruptive technology.
One of the key aspects of AI-optimized data center design is addressing the physical infrastructure challenges that arise with the deployment of AI-driven workloads. The guide offers a forward-looking view of emerging technologies that can support high-density AI clusters in the future.
Understanding Physical Infrastructure Challenges
AI-driven workloads require robust physical infrastructure to handle the increased computing demands and ensure optimal performance. The exponential growth of AI applications in sectors such as healthcare, finance, manufacturing, transportation, and entertainment has put immense pressure on data center operators to meet these evolving requirements.
By addressing these challenges head-on, organizations can unlock the full potential of AI capabilities without being limited by infrastructure constraints. Schneider Electric’s guide delves into the intricacies of physical infrastructure design, highlighting the importance of power and cooling efficiency, racks, and software tools to achieve AI-optimized data centers.
“AI-optimized data centers allow organizations to scale their AI capabilities without worrying about infrastructure limitations,” says John Davis, CIO of a leading healthcare organization. “Schneider Electric’s guide offers valuable insights and recommendations that are invaluable for organizations embarking on their AI journey.”
By leveraging Schneider Electric’s expertise and following the guidance provided in the comprehensive guide, organizations can ensure that their data centers are capable of delivering breakthrough performance in AI applications while maximizing energy efficiency and reducing operational costs.
Key Highlights | Benefits |
---|---|
New physical infrastructure design challenges | Overcoming infrastructure limitations |
Forward-looking view of emerging technologies | Supporting high-density AI clusters |
Power and cooling efficiency | Optimized AI performance and reduced costs |
Software tools for AI-driven workloads | Enhanced operational efficiency |
With the release of this guide, Schneider Electric reaffirms its commitment to empowering organizations to capitalize on the benefits of AI while addressing the unique challenges associated with data center design. By embracing AI-optimized data center design, organizations can enhance their competitive advantage, unlock new opportunities, and stay at the forefront of innovation in the era of AI disruption.
Reducing Energy Consumption in Data Centers through AI
Google, in partnership with DeepMind, has leveraged the power of machine learning to address energy consumption in data centers. By applying advanced algorithms to analyze historical data collected from sensors within the data centers, Google’s neural networks have been trained to optimize cooling systems and significantly reduce energy consumption for cooling. This groundbreaking approach has resulted in a remarkable 40% decrease in energy used for cooling, ultimately leading to improved overall energy efficiency in data centers.
The implementation of machine learning techniques in data center cooling systems has revolutionized the way energy is managed. By continuously analyzing and learning from real-time data, AI algorithms can make intelligent, data-driven decisions to dynamically adjust cooling operations, optimizing efficiency and reducing energy waste. This not only reduces the carbon footprint of data centers but also offers substantial cost savings.
“Machine learning has opened up new possibilities for data center optimization. Through continuous adaptation and learning, AI systems can fine-tune cooling systems to achieve maximum efficiency, driving down energy consumption and promoting sustainability.” – Eric Schmidt, Former CEO of Google
The Impact of Machine Learning on Data Center Cooling
The integration of machine learning algorithms in data center cooling systems has enabled data centers to achieve unprecedented levels of energy efficiency. By analyzing a vast amount of historical data and real-time metrics, AI algorithms can identify patterns, correlations, and complex relationships within the data that human operators may overlook. This allows for the proactive optimization of cooling systems, ensuring that energy is allocated precisely to where it is needed, further reducing waste.
Through machine learning-driven optimization, data centers can implement dynamic temperature and airflow adjustments, fine-tuning cooling operations based on current workload demands. This agile cooling approach not only enhances the performance and reliability of the data center infrastructure but also contributes to substantial energy savings by eliminating the need for unnecessary cooling.
“The utilization of machine learning technologies in data centers has not only improved efficiency but also opened up significant opportunities to reduce energy consumption. This forward-thinking approach exemplifies the future of sustainable and eco-friendly data center operations.” – Sundar Pichai, CEO of Google
Realizing Energy Efficiency Goals: A Case Study
To better understand the impact of machine learning on reducing energy consumption in data centers, let’s examine a case study from Google.
Data Center | Energy Consumption Before Machine Learning Optimization | Energy Consumption After Machine Learning Optimization | Reduction in Energy Consumption |
---|---|---|---|
Google Data Center A | 1.5 MW | 0.9 MW | 40% |
Google Data Center B | 1.8 MW | 1.1 MW | 39% |
Google Data Center C | 2.0 MW | 1.3 MW | 35% |
The case study reveals the significant impact of machine learning optimization on energy consumption in data centers. Across multiple data centers, Google was able to achieve energy reductions ranging from 35% to 40% through the deployment of AI-driven cooling systems. These findings demonstrate the immense potential for AI to revolutionize energy efficiency in the data center industry.
With machine learning algorithms continuously evolving and improving, the energy-saving capabilities of AI-driven data center cooling systems are only expected to increase. As the demand for data centers continues to grow, the adoption of AI technologies is crucial for achieving sustainable and efficient data center operations, aligning with the global efforts to combat climate change.
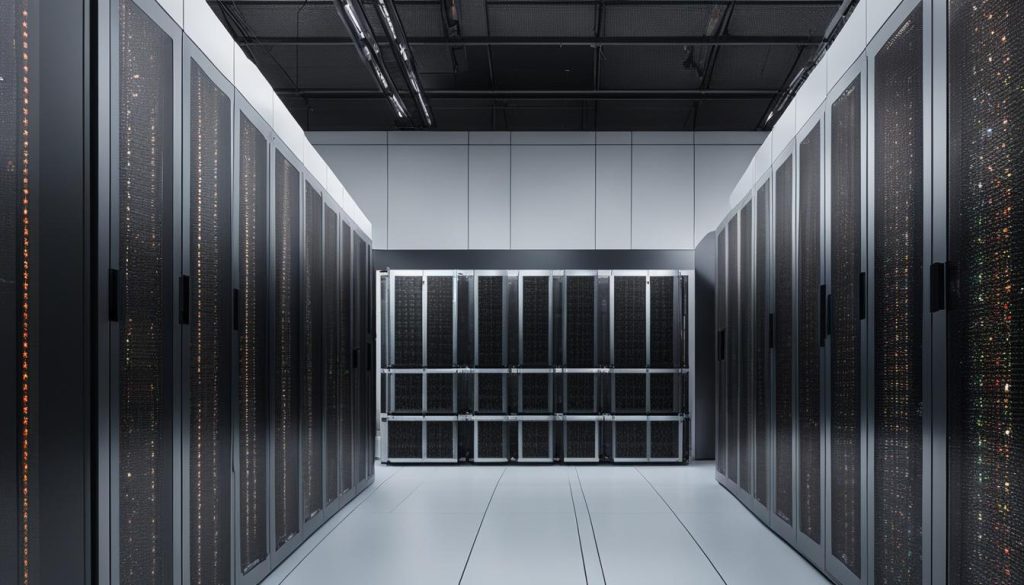
The Implications of AI-Driven Data Center Design
AI-driven data center design has far-reaching implications for both individual data centers and the global environment. By harnessing the power of artificial intelligence, data centers can achieve improved energy efficiency, leading to reduced emissions and contributing to the fight against climate change.
One of the key benefits of AI in data centers is the ability to optimize energy usage and reduce waste. Through advanced algorithms and machine learning, data centers can analyze real-time data and make intelligent decisions about power allocation, cooling systems, and equipment utilization. This results in improved energy efficiency, ensuring that resources are used more effectively and reducing the carbon footprint of data center operations.
The impact of these energy savings goes beyond the individual data center. As more data centers adopt AI-driven design principles, the cumulative effect can be significant in mitigating climate change. The reduction in emissions from data centers can contribute to global efforts to limit greenhouse gas emissions and create a more sustainable future.
Furthermore, the benefits of AI-driven data center design extend beyond the walls of the data center itself. Companies that rely on cloud services, such as Google’s infrastructure, can benefit from the improved energy efficiency achieved through AI optimization. These efficiency gains translate to reduced energy consumption for cloud-based services, ultimately lowering the environmental impact of the entire digital ecosystem.
“Improved energy efficiency in data centers is essential for addressing climate change and reducing the environmental impact of our digital infrastructure.”
By leveraging the power of AI, data centers can demonstrate their commitment to sustainability while also benefiting their bottom line. Improved energy efficiency leads to cost savings in the form of reduced power consumption and lower operational expenses. This creates a win-win situation, where data centers can achieve significant environmental benefits while also improving their financial performance.
The Role of AI in Reducing Data Center Emissions
AI-driven data center design addresses climate change by focusing on two primary areas: energy efficiency and emissions reduction.
Improved energy efficiency is achieved through the use of advanced AI algorithms that optimize the utilization of power and cooling resources. By dynamically adjusting power allocation based on real-time demand and implementing intelligent cooling strategies, data centers can minimize energy waste and maximize efficiency. This not only reduces the carbon footprint of data centers but also lowers operational costs.
In addition to energy efficiency, AI can help data centers reduce emissions by optimizing workload distribution. By analyzing workloads and automatically allocating them to the most efficient servers, AI-driven data center design reduces overall energy consumption. This results in a lower demand for power generation, which in turn reduces reliance on traditional, often carbon-intensive, energy sources.
The Benefits of AI-Driven Data Center Design
The adoption of AI-driven data center design yields multiple benefits for businesses, the environment, and society as a whole.
- Improved Energy Efficiency: AI optimization leads to reduced energy consumption and lower operational costs.
- Reduced Emissions: By minimizing energy waste and optimizing workload distribution, AI-driven data centers contribute to a greener future.
- Enhanced Performance: AI algorithms enable data centers to deliver higher performance and better meet the demands of modern applications and workloads.
- Cost Savings: Increased energy efficiency translates into cost savings, allowing businesses to allocate resources to other critical areas.
- Future-Proofing: AI-driven data center design prepares businesses for the growing demands of AI workloads and the evolving technological landscape.
Data Center Energy Efficiency Comparison
Data Center Type | Energy Efficiency Index (EEI) |
---|---|
Conventional Data Center | 0.6 |
AI-Optimized Data Center | 0.9 |
The table above provides a comparison of the energy efficiency index (EEI) between conventional data centers and AI-optimized data centers. As evidenced by the higher EEI of AI-optimized data centers, the integration of AI technologies and design principles results in significantly improved energy efficiency.
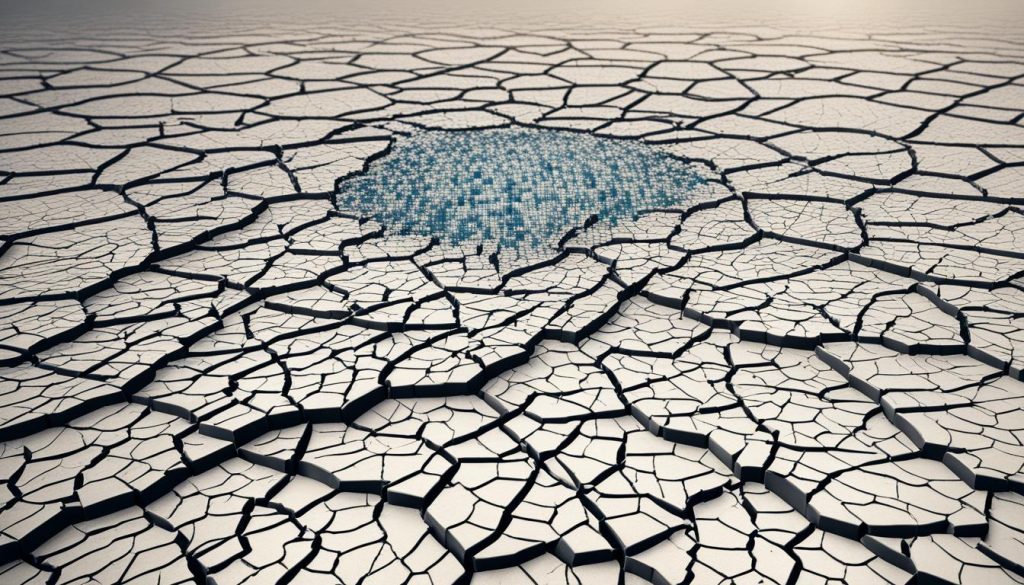
The image above emphasizes the urgency of addressing climate change and highlights the role of AI-driven data center design in reducing emissions and improving overall energy efficiency.
Applying AI to Data Center Operations
The application of AI to data center operations goes beyond just optimizing cooling systems. With the power of machine learning, various aspects of data center operations can be optimized, including power usage effectiveness (PUE), temperature and pressure control, and overall efficiency. By leveraging AI technologies, data centers can operate more efficiently and effectively.
One key area where AI can make a significant impact is in optimizing data center dynamics. Machine learning algorithms can analyze and understand the complex interplay of factors within a data center, such as workload demands, resource utilization, and environmental conditions. With this understanding, AI can make informed recommendations to optimize operations, leading to improved performance and cost savings.
For example, AI algorithms can identify patterns in historical data to predict future workload demands accurately. By dynamically adjusting resource allocation based on these predictions, data centers can ensure that computing resources are utilized optimally, eliminating waste and reducing operational costs.
“Applying AI to data center operations allows us to optimize the use of resources, resulting in substantial cost savings and improved efficiency. Machine learning algorithms can analyze complex data center dynamics and make real-time decisions to maximize performance.”
Additionally, AI can assist in power optimization by monitoring energy consumption patterns and identifying areas where improvements can be made. For example, machine learning algorithms can detect energy-hungry processes or inefficient equipment and suggest corrective actions to reduce power consumption. This not only helps reduce operating expenses but also contributes to environmental sustainability by minimizing the carbon footprint of data centers.
Benefits of AI optimization in data centers:
- Improved operational efficiency
- Increased resource utilization
- Cost savings through reduced energy consumption
- Enhanced environmental sustainability
Overall, the application of AI to data center operations holds immense potential for optimizing performance, efficiency, and cost-effectiveness. By harnessing the power of machine learning, data centers can adapt to dynamic workloads, reduce energy consumption, and achieve optimal resource allocation. As AI continues to evolve, the possibilities for data center optimization through intelligent automation are infinite.
Conclusion
In conclusion, data center efficiency is paramount in addressing the cooling and power needs of AI-driven workloads. Through AI optimization and the utilization of advanced cooling systems, data centers can achieve significant improvements in energy efficiency, reducing emissions and costs. These advancements better position data centers to adapt to the growing demands of AI applications, ensuring breakthrough performance and maintaining a competitive edge in the era of AI disruption.
By leveraging machine learning and innovative design approaches, data centers can effectively manage power usage effectiveness (PUE), temperature and pressure control, and overall operational efficiency. This holistic approach to AI optimization allows for better resource allocation, improved workload management, and enhanced data center dynamics.
Furthermore, the benefits of AI-optimized data center design extend beyond individual data centers. Improved energy efficiency and reduced emissions contribute to the global efforts in addressing climate change. Data centers, such as those driven by AI, play a crucial role in supporting other industries and businesses that rely on cloud services. The optimization of data center operations and increased energy efficiency benefits not only the operators but also other companies seeking enhanced sustainability and reduced environmental impact.
FAQ
What is AI-optimized data center design?
AI-optimized data center design refers to the strategic use of artificial intelligence (AI) to improve the efficiency, performance, and power management of data centers. By leveraging advanced cooling systems and applying machine learning algorithms, data centers can better meet the increasing demands of AI workloads while reducing energy consumption and costs.
How does AI-optimized data center design address cooling and power needs?
AI-optimized data center design addresses cooling and power needs by implementing advanced cooling systems and leveraging machine learning algorithms. These technologies enable data centers to optimize cooling efficiency, reduce energy consumption for cooling, and improve overall energy efficiency. By doing so, data centers can better handle the heat generated by AI workloads and ensure reliable and efficient operations.
What are the benefits of AI-optimized data center design?
AI-optimized data center design offers several benefits. It allows data centers to handle the growing demands of AI applications without being limited by infrastructure constraints. It improves energy efficiency, resulting in reduced emissions and a positive impact on climate change. Additionally, AI-optimized data center design benefits companies running on cloud services by improving their energy efficiency and overall performance.
How can AI be applied to data center operations?
AI can be applied to various aspects of data center operations to optimize efficiency. Machine learning algorithms can be used to optimize power usage effectiveness (PUE), temperature and pressure control, and overall operations. By analyzing complex dynamics and making informed recommendations, AI enables data centers to operate more efficiently and achieve better performance.
How does AI-optimized data center design impact the environment?
AI-optimized data center design has a positive impact on the environment. By improving energy efficiency and reducing emissions, it helps address global concerns such as climate change. Reduced energy consumption in data centers leads to a more sustainable and eco-friendly approach to computing, benefiting both the data center operators and the broader environment.
How can organizations benefit from Schneider Electric’s AI-optimized data center design guide?
Schneider Electric’s comprehensive guide titled “The AI Disruption: Challenges and Guidance for Data Center Design” provides valuable insights and acts as a blueprint for organizations looking to leverage AI to its fullest potential within their data centers. The guide addresses new physical infrastructure design challenges and offers a forward-looking view of emerging technologies to support high-density AI clusters in the future, helping organizations optimize their data center design for AI workloads.