Welcome to our article on U-Net, the groundbreaking architecture transforming the field of medical image segmentation.
Medical image segmentation plays a crucial role in precise diagnoses and treatment planning by identifying regions of interest within medical images.
The U-Net architecture combines the power of Vision Transformer (ViT) and U-Net models to achieve unmatched precision and efficiency in this process.
By leveraging global contextual analysis from ViT and local feature extraction from U-Net, the U-Net architecture provides high-resolution segmentation to accurately delineate anatomical structures and detect pathological abnormalities.
The operational mechanism of U-Net involves dissecting the image into patches and processing them through the transformer mechanism, followed by refinement using the U-Net architecture.
ViTransUNet, a specialized neural network architecture based on U-Net, has especially proven effective in medical imaging tasks like MRI, CT scans, and X-rays.
With its potential applications extending beyond medical imaging to satellite image analysis, autonomous vehicle navigation, and biological research, U-Net is revolutionizing not just one field but many.
Understanding Medical Image Segmentation
Medical image segmentation plays a pivotal role in the field of healthcare, enabling precise analysis of **anatomical structures** and **pathological abnormalities**. This process involves partitioning a medical image into distinct regions of interest to isolate and identify specific areas for detailed examination.
Accurate medical image segmentation is crucial for various applications, including precise diagnoses, treatment planning, and disease monitoring across different medical domains. By separating the image into different regions of interest, healthcare professionals can focus their attention on specific areas of concern, ensuring a comprehensive evaluation of the patient’s condition.
There are three main types of medical image segmentation techniques: manual, semi-automatic, and automatic. Manual segmentation involves experts meticulously **delineating regions of interest**, relying on their expertise and knowledge to identify relevant anatomical structures or pathological abnormalities.
Semi-automatic segmentation combines automated processes with manual refinement. It leverages advanced algorithms to generate an initial segmentation and allows experts to review and make necessary adjustments, ensuring accuracy and precision.
Automatic segmentation, on the other hand, relies on cutting-edge technologies such as deep learning to automatically identify and delineate regions of interest without human intervention. These advanced algorithms analyze the image data and classify different regions based on specific characteristics, making the process more efficient and less time-consuming.
Medical image segmentation is a complex and challenging task, but advancements in technology, especially in the field of deep learning, have significantly improved its accuracy and efficiency. With the aid of convolutional neural networks (CNNs), it is now possible to extract intricate features from medical images and accurately identify **regions of interest**.
Accurate medical image segmentation provides valuable insights and assists in the precise diagnosis of diseases, treatment planning, and the monitoring of pathological abnormalities. It enables healthcare professionals to make informed decisions and deliver personalized care to patients.
To better understand the significance of medical image segmentation, consider the following table that highlights its key applications across different medical domains:
Medical Domain | Applications |
---|---|
Radiology | Identifying tumors and lesions |
Pathology | Quantifying tissue characteristics |
Cardiology | Assessing cardiac function and morphology |
Neurology | Detecting brain abnormalities and lesions |
Oncology | Monitoring tumor growth and response to therapy |
Medical image segmentation plays a critical role in advancing healthcare practices by enabling precise analysis and diagnosis. With its ability to identify and isolate **anatomical structures** and **pathological abnormalities**, it has become an indispensable tool for healthcare professionals in delivering effective and personalized care to patients.
The Rise of Deep Learning in Medical Image Segmentation
Deep learning has revolutionized medical image segmentation, particularly with the use of convolutional neural networks (CNNs). CNNs excel at extracting intricate patterns and features from medical images, surpassing traditional segmentation methods. The UNet architecture, introduced in 2015, has played a significant role in the rise of deep learning in medical image segmentation.
Deep learning algorithms offer advantages over traditional methods, such as automated feature extraction and scalability. The UNet architecture, with its U-shaped design and skip connections, addresses challenges in medical image segmentation, retaining fine-grained details and capturing local and global context. Variants of the UNet architecture, such as UNet++, UNet 3+, and Attention UNet, further enhance its performance in specific segmentation tasks.
Deep learning in medical image segmentation extends beyond its primary application. It has proved valuable in disease diagnosis, quantitative image analysis, and multimodal fusion. Leveraging the power of deep learning, healthcare professionals can accurately identify and classify various diseases, analyze images quantitatively for objective evaluation, and combine data from different imaging modalities to gain comprehensive insights.
Advantages of Deep Learning in Medical Image Segmentation:
- Automated feature extraction
- Scalability
- Retains fine-grained details
- Captures local and global context
“Deep learning algorithms excel at extracting intricate patterns and features from medical images.”
– Expert in Medical Image Segmentation
The integration of deep learning and the UNet architecture has reshaped the medical imaging landscape, offering unparalleled precision and efficiency in image segmentation. These advancements have significantly impacted disease diagnosis, treatment planning, and quantitative analysis, ultimately improving patient care outcomes.
Advantages of Deep Learning in Medical Image Segmentation | Applications |
---|---|
Automated feature extraction | Disease diagnosis |
Scalability | Quantitative image analysis |
Retains fine-grained details | Multimodal fusion |
Captures local and global context |
Deep learning and the UNet architecture continue to propel the field of medical image segmentation forward, unlocking new possibilities for research, diagnosis, and treatment. The combination of deep learning’s capabilities and the adaptability of the UNet architecture ensures the ongoing development and enhancement of medical imaging techniques.
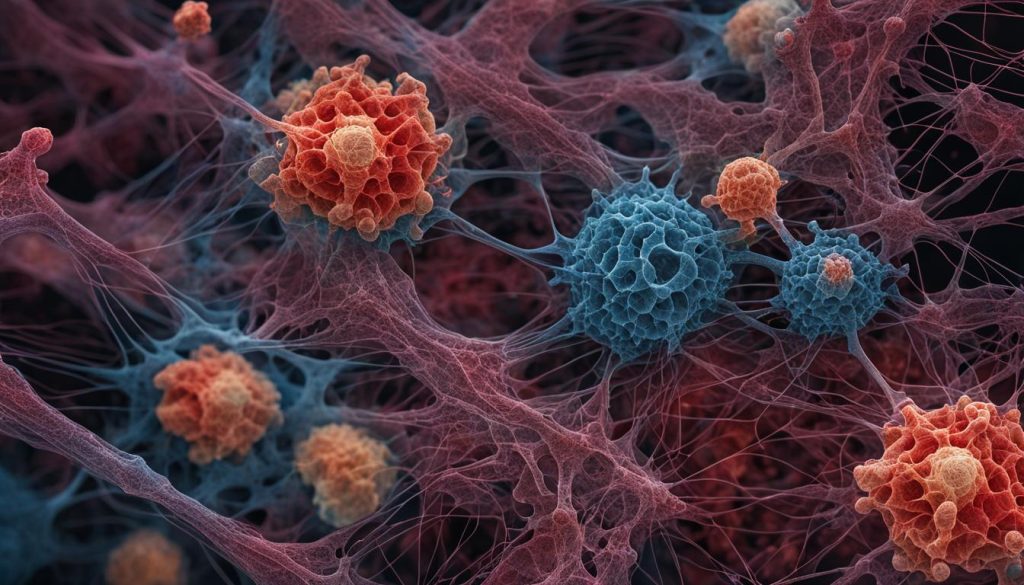
Advantages and Applications of Deep Learning in Medical Image Analysis
Deep learning has emerged as a powerful tool in medical image analysis, offering a multitude of advantages for accurate and efficient diagnoses, treatment planning, and quantitative analysis. By leveraging deep neural networks, medical professionals can extract meaningful features from images, leading to improved accuracy, precision, and overall patient outcomes.
One of the key strengths of deep learning lies in disease diagnosis and classification. Deep neural networks excel at learning complex patterns and recognizing subtle indicators of diseases, enabling them to categorize conditions with a high level of accuracy. This capability proves particularly valuable in cases where early detection is crucial.
Another area where deep learning excels is image segmentation and localization. By effectively separating different structures or abnormalities within medical images, deep learning algorithms facilitate precise diagnoses and aid in treatment planning. This capability allows medical professionals to accurately identify regions of interest, facilitating targeted interventions.
Quantitative image analysis is another field where deep learning plays a vital role. By extracting measurements and biomarkers automatically, deep learning models provide an objective evaluation of diseases. This feature is particularly useful in monitoring disease progression, assessing treatment responses, and facilitating data-driven decision-making.
Deep learning also enables multimodal fusion and integration in medical image analysis. By combining data from various imaging modalities, such as MRI, CT scans, and PET scans, deep learning algorithms facilitate a comprehensive understanding of a patient’s condition. This holistic approach can lead to more informed diagnoses and treatment plans.
“Deep learning algorithms offer unparalleled accuracy and efficiency in medical image analysis, transforming the way we approach disease diagnosis, treatment planning, and quantitative analysis.”
These advancements in deep learning have led to successful applications across multiple medical domains. In radiology, deep learning algorithms have been used to automate the detection of abnormalities, reducing the burden on radiologists and enabling faster diagnoses. In pathology, deep learning models assist in analyzing histopathological images, aiding in cancer detection and grading. In surgery, deep learning plays a crucial role in surgical planning and real-time intraoperative guidance, contributing to improved surgical outcomes.
With its ability to combine accuracy, efficiency, and automation, deep learning has become an indispensable tool in medical image analysis. By harnessing the power of deep neural networks, healthcare providers can enhance patient care, improve diagnostic capabilities, and advance medical research.
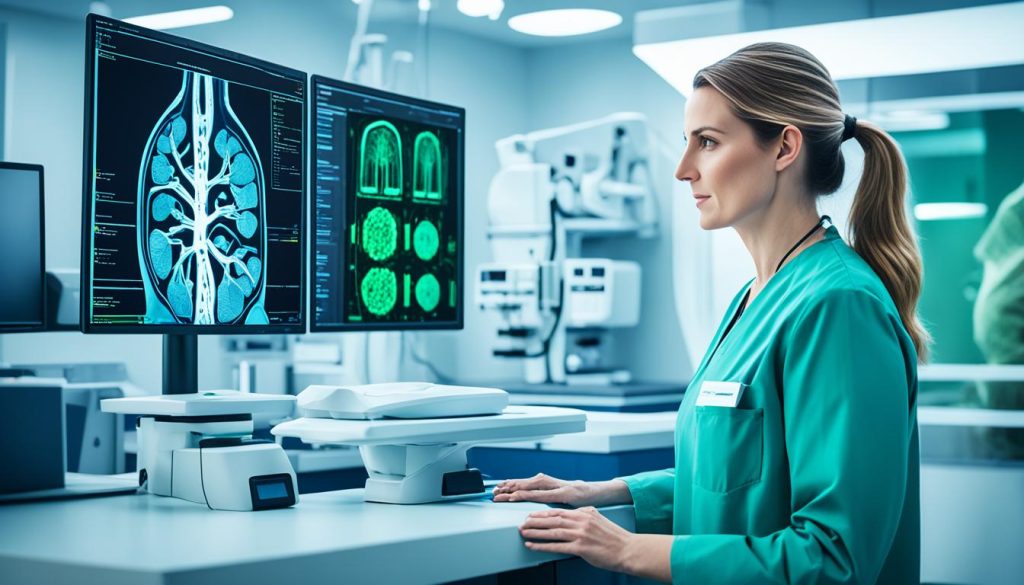
Advantages of Deep Learning in Medical Image Analysis | Applications of Deep Learning in Medical Image Analysis |
---|---|
|
|
Conclusion
The U-Net architecture has revolutionized medical image segmentation, ushering in a new era of precision and efficiency in the field of medical imaging. By leveraging the combined strengths of Vision Transformer and U-Net, this innovative architecture offers unparalleled accuracy and optimization.
Deep learning, particularly through the use of convolutional neural networks (CNNs), has played a pivotal role in advancing medical image segmentation. These powerful algorithms provide a level of accuracy, efficiency, and automation that surpasses traditional methods, enabling more precise and objective analysis of medical images.
With the rise of deep learning in medical image segmentation, significant advancements have been achieved in disease diagnosis, treatment planning, and quantitative analysis. The integration of deep learning and the U-Net architecture continues to drive innovation in medical imaging, unlocking new possibilities for enhanced patient care and groundbreaking medical research.
FAQ
What is the U-Net architecture?
The U-Net architecture is a groundbreaking innovation in the field of medical image segmentation. It combines the strengths of Vision Transformer (ViT) and U-Net models to offer unparalleled precision and efficiency.
How does the U-Net architecture work?
The operational mechanism of U-Net involves dissecting the image into patches and processing them through the transformer mechanism, followed by refinement using the U-Net architecture. ViT provides a global understanding of the image, while U-Net enables local feature extraction and high-resolution segmentation.
What is ViTransUNet?
ViTransUNet is a specialized neural network architecture based on U-Net. It is particularly useful for medical imaging tasks such as MRI, CT scans, and X-rays. The integration of ViT and U-Net in ViTransUNet brings together global contextual analysis and local precision to accurately identify and delineate structures within medical images.
What is medical image segmentation?
Medical image segmentation is the process of partitioning a medical image into distinct regions of interest, enabling precise analysis of anatomical structures and pathological abnormalities. The purpose of medical image segmentation is to isolate and identify specific regions within the image for detailed analysis.
What are the three main types of medical image segmentation techniques?
The three main types of medical image segmentation techniques are manual, semi-automatic, and automatic. Manual segmentation involves experts meticulously delineating regions of interest, while semi-automatic segmentation combines automated processes with manual refinement. Automatic segmentation uses advanced algorithms, like deep learning, to automatically identify and delineate regions without human intervention.
Why is accurate medical image segmentation important?
Accurate medical image segmentation is crucial for precise diagnoses, treatment planning, and disease monitoring in various medical domains. It enables healthcare professionals to analyze anatomical structures, identify pathological abnormalities, and make informed decisions regarding patient care.
What is deep learning’s role in medical image segmentation?
Deep learning has revolutionized medical image segmentation, particularly with the use of convolutional neural networks (CNNs). CNNs excel at extracting intricate patterns and features from medical images, surpassing traditional segmentation methods. The UNet architecture, introduced in 2015, has played a significant role in the rise of deep learning in medical image segmentation.
What are the advantages and applications of deep learning in medical image analysis?
Deep learning algorithms offer advantages over traditional methods, such as automated feature extraction and scalability. In medical image analysis, deep learning provides high accuracy, precision, and efficiency. It excels in disease diagnosis and classification, precise image segmentation and localization, quantitative image analysis, and multimodal fusion. Deep learning has applications in various medical domains, enhancing patient care and improving outcomes.
How has the rise of deep learning impacted medical image segmentation?
The rise of deep learning in medical image segmentation has led to remarkable advancements in disease diagnosis, treatment planning, and quantitative analysis. Deep learning algorithms, especially with the UNet architecture, provide accuracy, efficiency, and automation in medical image analysis, surpassing traditional methods. This integration continues to drive innovation in medical imaging, unlocking new possibilities for patient care and medical research.
How has the U-Net architecture transformed medical image segmentation?
The U-Net architecture has revolutionized medical image segmentation by combining the strengths of Vision Transformer and U-Net, offering unparalleled precision and efficiency. Its U-shaped design with skip connections addresses challenges in retaining fine-grained details and capturing local and global context. Variants of the UNet architecture further enhance its performance in specific segmentation tasks, leading to improved diagnoses and treatment planning.